Segmental online support vector regression method applied in traffic prediction
A support vector regression and traffic volume technology, which is applied in wireless communication, instruments, computing models, etc., can solve problems such as limited data history knowledge or models, decreased algorithm running speed, and decreased algorithm generalization ability, so as to avoid incremental The effect of the decline of online learning efficiency, the improvement of algorithm efficiency, the improvement of execution efficiency and prediction accuracy
- Summary
- Abstract
- Description
- Claims
- Application Information
AI Technical Summary
Problems solved by technology
Method used
Image
Examples
specific Embodiment approach 1
[0070] A piecewise online support vector regression method applied to traffic time series forecasting:
[0071] Definition: Online support vector regression model Online SVR, penalty parameter C, insensitive loss coefficient ε, kernel function type KernelType, kernel function parameter p, sub-segmentation model SOSVR(s), s=1, 2, 3..., Initial training set length TrainLength, segmentation condition SGP, selection of optimal sub-segmentation model prediction output condition SBPP, segmentation update mechanism UpdateSOSVR(l), l∈(1, 2, ..., s), embedding dimension EmbededDimension ;
[0072] Output: i-th step forecast value PredictL(i), time series real value Test(i);
[0073] Such as image 3 and Figure 4 Shown, method of the present invention comprises the steps:
[0074] Step 1. Data preprocessing: transform the time series data and perform phase space reconstruction to make it conform to the set embedded dimension EmbededDimension;
[0075] Step 2, Online SVR initializa...
specific Embodiment approach 2
[0088] Specific embodiment 2: In this embodiment, the segmentation condition SGP described in step 4 is used to ensure that the segmentation condition can save the historical knowledge of the data set with maximum efficiency, so that the difference of each sub-segment SOSVR model is maximized, and the model is enhanced. Generalization. Other steps are the same as in the first embodiment.
specific Embodiment approach 3
[0089] Embodiment 3: In step 4 of this embodiment, a clustering method is used as a segmentation condition, so that each sub-segment SOSVR(s) adapts to sub-time series segments with different characteristics. Other steps are the same as those in Embodiment 1 or 2.
PUM
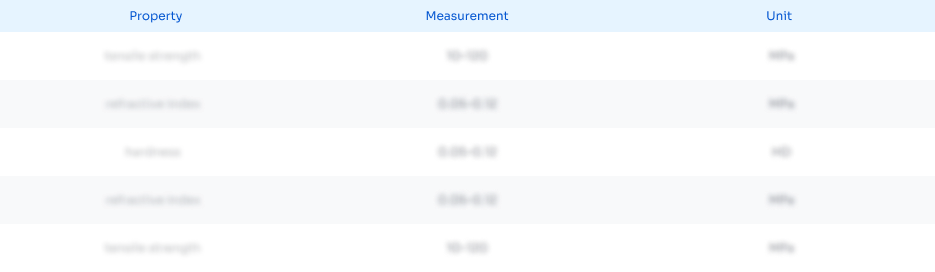
Abstract
Description
Claims
Application Information
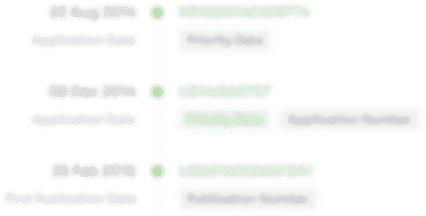
- R&D Engineer
- R&D Manager
- IP Professional
- Industry Leading Data Capabilities
- Powerful AI technology
- Patent DNA Extraction
Browse by: Latest US Patents, China's latest patents, Technical Efficacy Thesaurus, Application Domain, Technology Topic, Popular Technical Reports.
© 2024 PatSnap. All rights reserved.Legal|Privacy policy|Modern Slavery Act Transparency Statement|Sitemap|About US| Contact US: help@patsnap.com