Semi-supervised mass data hierarchy classification method
A mass data and classification method technology, applied in the direction of electrical digital data processing, special data processing applications, instruments, etc., can solve the problems of time and cost, and the inability to establish classification models for mass text data, and achieve the effect of expanding the scale
- Summary
- Abstract
- Description
- Claims
- Application Information
AI Technical Summary
Problems solved by technology
Method used
Image
Examples
Embodiment Construction
[0036] A semi-supervised hierarchical classification method for massive data, which uses a semi-supervised learning method (semi-supervised learning) to reduce the workload of manually labeling the training set, and proposes a random progressive method to train regular linear classifiers, so that Classifiers can use massive text data to train and produce high-precision classification models.
[0037] The basic idea of the present invention is to set up a classifier for each node (non-root node) in the hierarchical structure to classify the webpages flowing through its parent node to its child nodes; Improve the classification effect; in the training process, we use the stochastic gradient descent (Stochastic gradient decent) method to traverse the massive training set multiple times, reducing the computational complexity to O(N), thus solving the problem of large-scale data set training question. The classification steps of this hierarchical classifier are as follows:
[0...
PUM
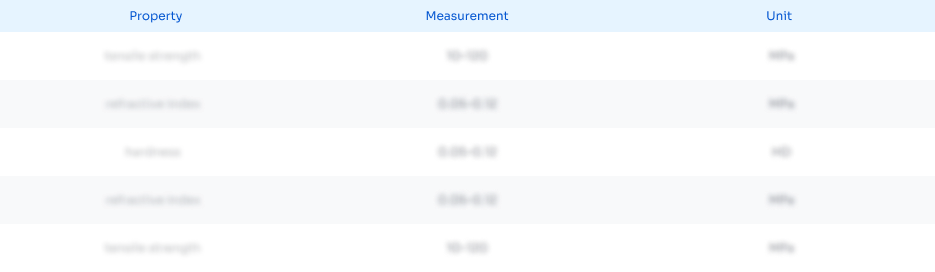
Abstract
Description
Claims
Application Information
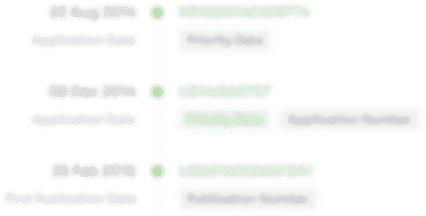
- R&D Engineer
- R&D Manager
- IP Professional
- Industry Leading Data Capabilities
- Powerful AI technology
- Patent DNA Extraction
Browse by: Latest US Patents, China's latest patents, Technical Efficacy Thesaurus, Application Domain, Technology Topic, Popular Technical Reports.
© 2024 PatSnap. All rights reserved.Legal|Privacy policy|Modern Slavery Act Transparency Statement|Sitemap|About US| Contact US: help@patsnap.com