Method for optimal maintenance decision-making of hydraulic equipment with risk control
A hydraulic equipment and optimal technology, applied in special data processing applications, instruments, biological neural network models, etc., can solve problems such as different status, improve accuracy, improve fault diagnosis accuracy and algorithm efficiency, and speed up diagnosis. Effect
- Summary
- Abstract
- Description
- Claims
- Application Information
AI Technical Summary
Problems solved by technology
Method used
Image
Examples
Embodiment 1
[0163] Embodiment 1: Using variable weight association rule algorithm DVWAR to calculate the probability value of the corresponding potential fault
[0164] In this embodiment, two methods are used for analysis, one is the weighted association rule algorithm, and the other is the variable weight association rule algorithm. The same set of data is used in the example, and the final results are obtained through calculation and compared. .
example
[0165] Example: The hydraulic equipment M is composed of 5 components, and there are 7 possible failures. Table 1 is the initial weight of each component, and Table 2 is the fault database, indicating the components that can be traced when a certain fault occurs. Set the minimum support threshold wminsup to 1.
[0166] Table 1 Initial weights of each component of equipment M Table 2 Each fault database of equipment M
[0167]
[0168] 1. Mining using weighted association rule algorithm
[0169] Since the weighted association rule algorithm is given to each component only once in the life cycle of the device, the initial weight of each component of the device M is constant, that is, the weight of component A is 0.2, and the weight of component B is 0.2. The weight is 0.1, the weight of component C is 0.3, the weight of component D is 0.4, and the weight of component E is 0.8. According to the mining method of weighted association rules, mining is carried out according to ...
Embodiment 2
[0180] Embodiment 2: Using neural network modeling to obtain the consequence value of the corresponding fault
[0181] In order to illustrate the application of BP neural network in the prediction of failure consequence value, five items of hydraulic equipment risk value, personal risk value, environmental risk value, social risk value and system risk value are selected as input items, and the output item is the comprehensive potential failure consequence value. Evaluation value. Due to the large data, Table 6 lists the learning samples for predicting the comprehensive evaluation value of some fault consequences.
[0182] Table 6 Learning samples for prediction of comprehensive evaluation value of partial failure consequences
[0183]
[0184] In the design of BP neural network, if the number of nodes in the hidden layer of the network is too small, the nonlinear mapping function and fault tolerance of the network will be poor. If the number of nodes is too large, the lear...
PUM
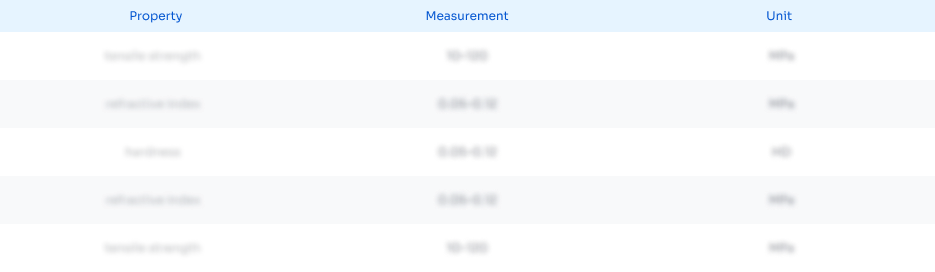
Abstract
Description
Claims
Application Information
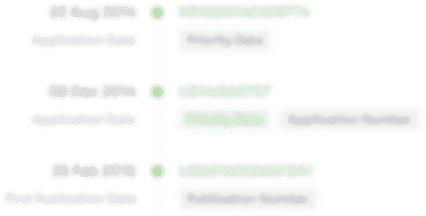
- R&D Engineer
- R&D Manager
- IP Professional
- Industry Leading Data Capabilities
- Powerful AI technology
- Patent DNA Extraction
Browse by: Latest US Patents, China's latest patents, Technical Efficacy Thesaurus, Application Domain, Technology Topic, Popular Technical Reports.
© 2024 PatSnap. All rights reserved.Legal|Privacy policy|Modern Slavery Act Transparency Statement|Sitemap|About US| Contact US: help@patsnap.com