Daily gas load combination prediction method based on generalized dynamic fuzzy neural network
A neural network and dynamic fuzzy technology, applied in biological neural network models, special data processing applications, instruments, etc., can solve the problems of insufficient regular processing, poor prediction accuracy, and the inability to conveniently consider weather conditions, etc., to achieve accurate sex high effect
- Summary
- Abstract
- Description
- Claims
- Application Information
AI Technical Summary
Problems solved by technology
Method used
Image
Examples
Embodiment Construction
[0077] The present invention is described in more detail below in conjunction with accompanying drawing example:
[0078] 1. Use the generalized regression neural network and gray neural network based on differential processing to predict the daily gas load.
[0079] The general steps are:
[0080] (1) Data preprocessing. That is, first carry out abnormal data judgment and processing (with reference to similar day data, similar day is the closest to it with similar weather, temperature, day of the week, etc.) before neural network training, and then the data are normalized, the present invention Normalize the data to the (0,1) interval;
[0081] (2) Select the network input and output nodes. Considering the periodicity and trend of the daily gas consumption, we choose the daily gas load value one day before the forecast date and seven days before the forecast date as input nodes, a total of two input nodes. Select the predicted daily gas load value as the output node;
[...
PUM
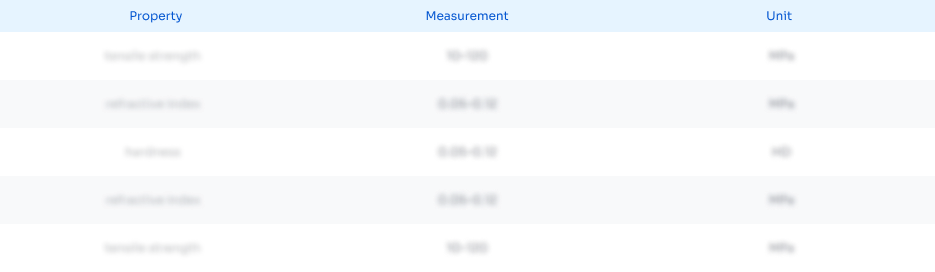
Abstract
Description
Claims
Application Information
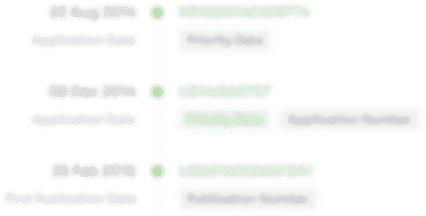
- Generate Ideas
- Intellectual Property
- Life Sciences
- Materials
- Tech Scout
- Unparalleled Data Quality
- Higher Quality Content
- 60% Fewer Hallucinations
Browse by: Latest US Patents, China's latest patents, Technical Efficacy Thesaurus, Application Domain, Technology Topic, Popular Technical Reports.
© 2025 PatSnap. All rights reserved.Legal|Privacy policy|Modern Slavery Act Transparency Statement|Sitemap|About US| Contact US: help@patsnap.com