Neural network-based low amplitude network flow anomaly detection method
A neural network and network traffic technology, applied in the field of abnormal detection of low-amplitude network traffic based on neural networks, can solve the problems that the OD flow traffic cannot be directly measured, the OD flow inversion results are inaccurate, and the detection steps are affected. Solve the effect of inversion error affecting the detection
- Summary
- Abstract
- Description
- Claims
- Application Information
AI Technical Summary
Problems solved by technology
Method used
Image
Examples
Embodiment Construction
[0019] The present invention will be further described below in conjunction with the accompanying drawings.
[0020] figure 1 A schematic diagram of the principle of the neural network module in the present invention is shown. Described neural network module comprises first and second multi-layer recursive neural network 2; The input of described second multi-layer recurrent neural network 2 is link flow L (m)={l that can be directly measured 1 (m), l 2 (m),...,l n (m)}, where n is the number of link traffic, m is the current sampling moment, l n (m) is the nth link flow; the output of the second multi-layer recursive neural network 2 is the partial OD flow estimation flow, and the partial OD flow estimation flow is expressed as The input of the first multi-layer recurrent neural network 1 is the link flow L(m) and the partial OD flow estimated flow as a supplementary constraint input, since the partial OD flow estimated flow is the second multi-layer recurrent neural net...
PUM
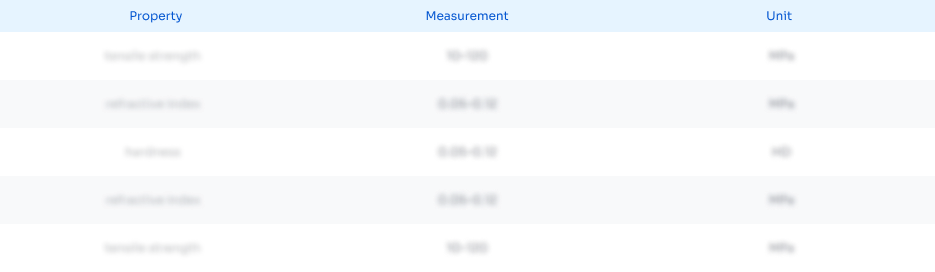
Abstract
Description
Claims
Application Information
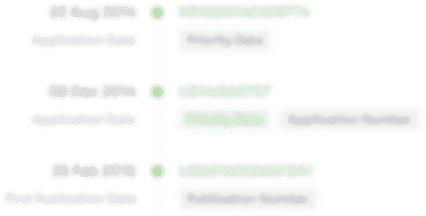
- R&D
- Intellectual Property
- Life Sciences
- Materials
- Tech Scout
- Unparalleled Data Quality
- Higher Quality Content
- 60% Fewer Hallucinations
Browse by: Latest US Patents, China's latest patents, Technical Efficacy Thesaurus, Application Domain, Technology Topic, Popular Technical Reports.
© 2025 PatSnap. All rights reserved.Legal|Privacy policy|Modern Slavery Act Transparency Statement|Sitemap|About US| Contact US: help@patsnap.com