Integrated transfer learning method for classification of unbalance samples
A transfer learning and unbalanced technology, applied in the field of machine learning, can solve problems such as classification accuracy decline, imbalance, and importance difference, and achieve the effect of improving efficiency and accuracy, and increasing contribution rate
- Summary
- Abstract
- Description
- Claims
- Application Information
AI Technical Summary
Problems solved by technology
Method used
Image
Examples
Embodiment Construction
[0046] The integrated migration learning method (referred to as UBITLA) of unbalanced sample classification provided by the present invention, the steps are as follows (refer to figure 1 ):
[0047] 1. Input: The input data comes from two parts: migration auxiliary data set A and target data set O. Part of the data is extracted from these two parts of data and mixed in proportion to form a training data set C={(X 1 , Y 1 ), (X 2 , Y 2 ),…, (X N , Y N )}, where (X i , Y i ) is a training sample composed of sample feature attribute vector and sample category. i=1, 2, . . . , N. The first n samples in C are the data in A, and the remaining m samples in C are the data in O (n+m=N). The predetermined number of iterations is T. where X i ∈X, X is the input sample data, X i Is the characteristic attribute vector of the sample, the dimension is q, Y i ∈{0,+1} is the class label of the sample.
[0048] 2. Initialize sample weights:
[0049]
[0050] in, is the ini...
PUM
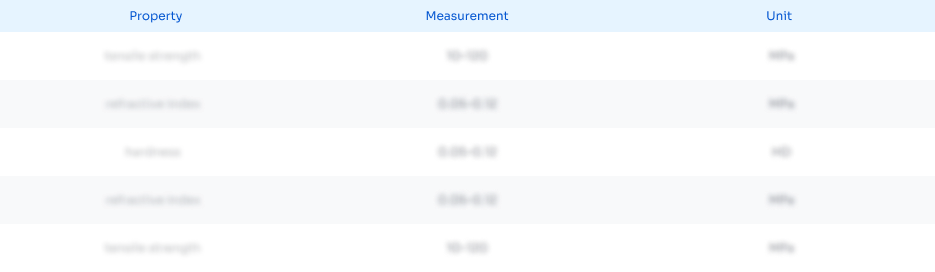
Abstract
Description
Claims
Application Information
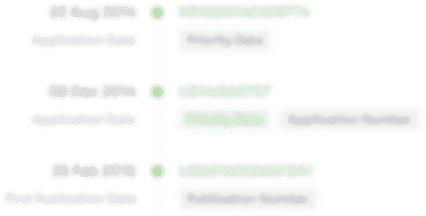
- R&D Engineer
- R&D Manager
- IP Professional
- Industry Leading Data Capabilities
- Powerful AI technology
- Patent DNA Extraction
Browse by: Latest US Patents, China's latest patents, Technical Efficacy Thesaurus, Application Domain, Technology Topic.
© 2024 PatSnap. All rights reserved.Legal|Privacy policy|Modern Slavery Act Transparency Statement|Sitemap