Multi-group image classification method based on characteristic expansion and fuzzy support vector machine
A technology of fuzzy support vector and classification method, applied in the field of image processing, can solve the problems of low classification accuracy and the inability of multi-group image classification method to effectively extract the essential features of images, so as to improve the classification accuracy and avoid the disaster of dimensionality.
- Summary
- Abstract
- Description
- Claims
- Application Information
AI Technical Summary
Problems solved by technology
Method used
Image
Examples
specific Embodiment approach 1
[0032] Specific implementation mode one: the following combination figure 1 Describe this embodiment, the multi-group image classification method based on feature extension and fuzzy support vector machine described in this embodiment, the method comprises the following steps:
[0033] Step 1. Initialize the given number of bands as I 0 , a multi-group image of size P×Q
[0034] IM j (p, q), j=1, 2, ..., I 0 , p=1, 2,..., P, q=1, 2,..., Q,
[0035] Remove multi-group image IM j (p, q) are severely polluted by noise and other bands that cannot be used, and the remaining I effective bands are reordered to obtain multi-group images to be expanded
[0036] IM i (p, q), i=1, 2, ..., 1,
[0037] where I 0 , I, P and Q are natural numbers;
[0038] Step 2, successively to I multi-group image IM to be extended i (p, q) perform two-dimensional empirical mode decomposition to obtain the I group of two-dimensional eigenmode functions
[0039] BIMF ...
specific Embodiment approach 2
[0052] Specific implementation mode two: the following combination figure 2 Describe this embodiment mode, this embodiment mode will be further described to embodiment mode one, in step 2 sequentially to 1 multi-packet image IM to be extended i (p, q) perform two-dimensional empirical mode decomposition to obtain the I group of two-dimensional eigenmode functions The process is:
[0053] Step A. Initialize r 1 =IM i (p,q); u=1; v=0; SD=1000; h u,v = r 1 ; c u = r 1 ,
[0054] Among them, the to-be-expanded multi-group image of the i-th band to be processed is IM i (p,q),
[0055] r 1 is the residual after the first two-dimensional empirical mode decomposition,
[0056] SD is the termination iteration threshold,
[0057] h u,v is the residual function after the vth screening in the uth two-dimensional empirical mode decomposition,
[0058] c u is the two-dimensional eigenmode function
[0059] Step B, let v=v+1; h u,(v-1) = r u , and by comparing with adja...
specific Embodiment approach 3
[0074] Specific implementation mode three: this implementation mode will further explain implementation mode one, and in step three, all two-dimensional eigenmode functions Organic combination, the process of obtaining the extended feature FBIMF is:
[0075] The i=1, 2, ..., the two-dimensional intrinsic mode function of the I band are processed in sequence, and the extended feature FBIMF corresponding to the two-dimensional intrinsic mode function of each band is obtained, and the two-dimensional intrinsic mode function of each band is The method of obtaining the extended characteristic FBIMF of the intrinsic mode function is the same, as follows: in the two-dimensional intrinsic mode function of the band i = 1, 2, ..., U i For a BIMF, if u i is an odd number, then the link directly to tail if u i is an even number, then the Flip left and right and connect to Tail, in turn, until all U of the two-dimensional intrinsic mode function of the band i All BIMFs are pro...
PUM
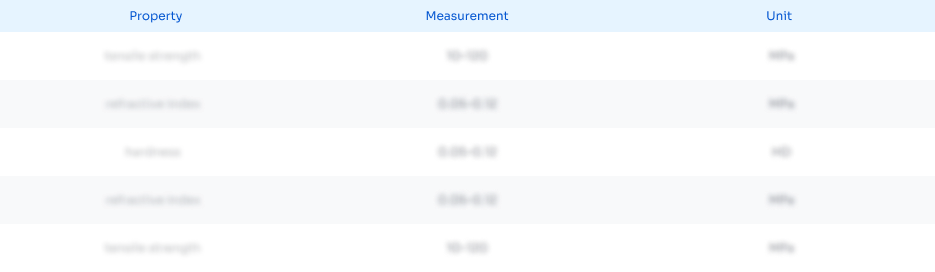
Abstract
Description
Claims
Application Information
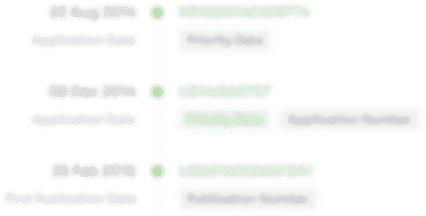
- R&D Engineer
- R&D Manager
- IP Professional
- Industry Leading Data Capabilities
- Powerful AI technology
- Patent DNA Extraction
Browse by: Latest US Patents, China's latest patents, Technical Efficacy Thesaurus, Application Domain, Technology Topic, Popular Technical Reports.
© 2024 PatSnap. All rights reserved.Legal|Privacy policy|Modern Slavery Act Transparency Statement|Sitemap|About US| Contact US: help@patsnap.com