Cross-media information analysis and retrieval method
An information analysis and cross-media technology, applied in the field of multimedia information data retrieval, can solve problems such as semantic gap, low retrieval efficiency, and incomparability
- Summary
- Abstract
- Description
- Claims
- Application Information
AI Technical Summary
Problems solved by technology
Method used
Image
Examples
Embodiment 1
[0087] Assuming that there are 10,000 images and 10,000 audio clips, 500 in each category, and 20 categories, 20 multimedia documents can be constructed, and each multimedia document contains 1,000 multimedia objects (500 images and 500 audio). First extract the SIFT features of all images, and represent each image as a set of 128-dimensional visual feature vectors, and then extract the MFCC features of all audio, and each segment of audio is represented as a set of 21-dimensional auditory feature vectors. Construct multimedia documents, classify the image-audio database, and generate a training set D={D 1 ,...,D c ,...,D N}., each multimedia document D c is a collection of images and audios of type C. The information of multimedia documents is known, and the MC-PLSA model is used to learn multimedia documents and their characteristics. The MC-PLSA model mainly uses EM expected maximum value and asymmetric learning method to learn related parameters. The user inputs the mu...
Embodiment 2
[0089] The user submits an audio clip of a tiger's call to query the first 20 results returned by the image. The retrieval process is as follows: When the user submits an audio clip of a tiger's call as a retrieval example, the system first performs a search based on the audio features of the audio clip. The model learns to find the topic probability distribution of the multimedia semantic space to which the segment belongs. Then, according to the cosine angle value between all multimedia objects in the database and the subject probability distribution of the query example as the similarity, four correlation matrices C are formed IA , C AI , C II , C AA . Then update the values of the four association matrices according to the propagation model, that is, update the similarity values between all multimedia objects in the database and the query examples. And calculate the average similarity value between the query example and multimedia documents of each category, and th...
PUM
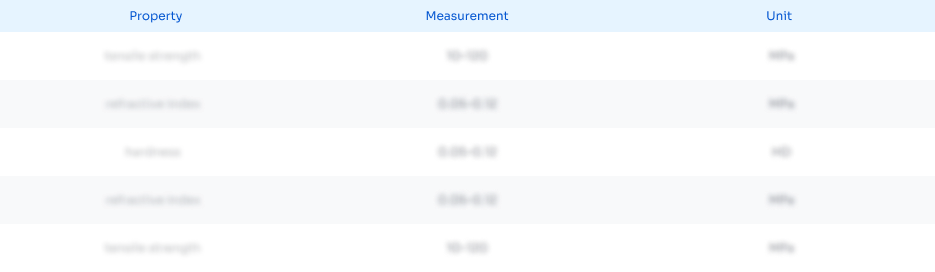
Abstract
Description
Claims
Application Information
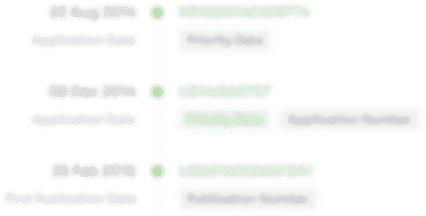
- R&D Engineer
- R&D Manager
- IP Professional
- Industry Leading Data Capabilities
- Powerful AI technology
- Patent DNA Extraction
Browse by: Latest US Patents, China's latest patents, Technical Efficacy Thesaurus, Application Domain, Technology Topic.
© 2024 PatSnap. All rights reserved.Legal|Privacy policy|Modern Slavery Act Transparency Statement|Sitemap