Enhanced relationship classifier based on representative samples
A relational classification and representative technology, applied in the field of pattern recognition, can solve the problems that the relational matrix R cannot correctly reflect the characteristics of data distribution, the classification lacks robustness, and cannot correctly and truly reflect the logical relationship between categories and structures.
- Summary
- Abstract
- Description
- Claims
- Application Information
AI Technical Summary
Problems solved by technology
Method used
Image
Examples
Embodiment Construction
[0030] The present invention has first set following experimental operating conditions:
[0031] 1. Each dimension feature in the data set is normalized to the interval [0, 1] through the maximum and minimum normalization method;
[0032] 2. The number of clusters c in EFRC is in [c min , c max ] determined within the range, where c min is the number of categories, c max for (N is the number of samples).
[0033] 3. X new The ratio λ to the original set X is chosen in the range (0,1).
[0034] Based on the above conditions, the enhanced relational classifier based on representative samples proposed by the present invention has been implemented in the scientific computing platform Matlab, and the effectiveness of the method is proved by the experimental results in Matlab.
[0035] The concrete method flowchart of EFRC classifier of the present invention is as figure 1 shown. Below in conjunction with accompanying drawing, further describe the specific implementation s...
PUM
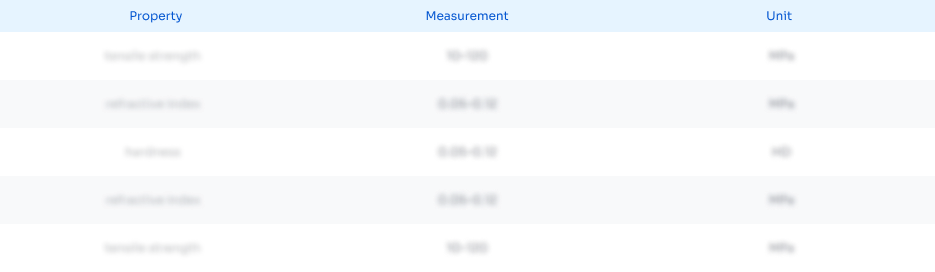
Abstract
Description
Claims
Application Information
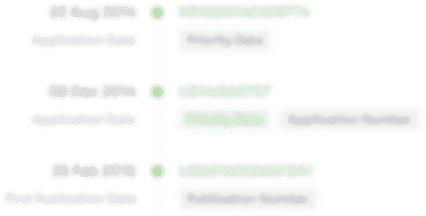
- R&D
- Intellectual Property
- Life Sciences
- Materials
- Tech Scout
- Unparalleled Data Quality
- Higher Quality Content
- 60% Fewer Hallucinations
Browse by: Latest US Patents, China's latest patents, Technical Efficacy Thesaurus, Application Domain, Technology Topic, Popular Technical Reports.
© 2025 PatSnap. All rights reserved.Legal|Privacy policy|Modern Slavery Act Transparency Statement|Sitemap|About US| Contact US: help@patsnap.com