Target tracking method based on semi-supervised learning and random fern classifier
A semi-supervised learning and fern classifier technology, applied in the field of target tracking, can solve problems such as adhesion, camera shake, and poor hyperplane rotation
- Summary
- Abstract
- Description
- Claims
- Application Information
AI Technical Summary
Problems solved by technology
Method used
Image
Examples
specific Embodiment approach 1
[0020] Specific implementation mode 1. Combination figure 1 Describe this embodiment, the target tracking method based on semi-supervised learning and random fern classifier. This method establishes a full-view online model of the target, obtains the position of the target through tracking, detection and its combination, and performs learning for the detector And online model update, this method is implemented by the following steps:
[0021] 1. Initialize the online model, scan the input image with S-shaped windows of different scales, keep the obtained windows whose size is larger than the threshold (thw=24), and calculate the overlap rate of each reserved window image with the initially selected target, Take the window image with the largest overlap rate as a positive example, randomly select several window images (n=100 ) as a negative example, the obtained positive and negative examples are added to the online model after image normalization;
[0022] 2. Initialize the ...
PUM
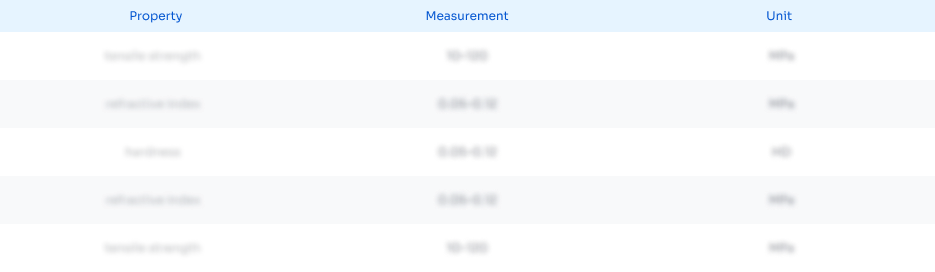
Abstract
Description
Claims
Application Information
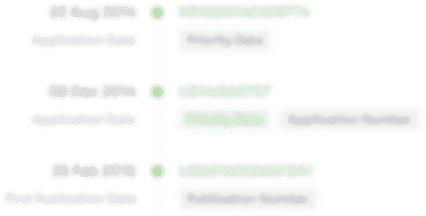
- R&D
- Intellectual Property
- Life Sciences
- Materials
- Tech Scout
- Unparalleled Data Quality
- Higher Quality Content
- 60% Fewer Hallucinations
Browse by: Latest US Patents, China's latest patents, Technical Efficacy Thesaurus, Application Domain, Technology Topic, Popular Technical Reports.
© 2025 PatSnap. All rights reserved.Legal|Privacy policy|Modern Slavery Act Transparency Statement|Sitemap|About US| Contact US: help@patsnap.com