Automatic micro-expression recognition method based on Gabor features and edge orientation histogram (EOH) features
A recognition method and micro-expression technology, applied in character and pattern recognition, instruments, computer components, etc., can solve the problems of high false alarm rate, low expression strength, incapable of micro-expression and macro-expression video segmentation, etc., to improve Speed and efficiency, the effect of improving recognition speed and accuracy
- Summary
- Abstract
- Description
- Claims
- Application Information
AI Technical Summary
Problems solved by technology
Method used
Image
Examples
Embodiment 1
[0037] Embodiment 1. Facial Expression Recognition Combining Gabor Features and Gentleboost
[0038] To be able to analyze micro-expressions in videos, it is first necessary to be able to recognize common expressions in still images. Embodiment 1 Firstly, the facial expression recognition performance of the algorithm is evaluated on the facial expression database (also known as CK).
[0039] The database contains 6 basic expression videos of 100 college students (age range: 18-30 years old). Among them, 65% of the models are women, 15% of the models are black, and 3% of the models are Asian or Latino. The video shooting method is: the model performs the prescribed facial expressions according to the requirements, and the camera records the frontal facial expressions of the subjects with an analog S-video signal. Videos are finally stored as 640×480 8-bit grayscale images.
[0040] In Example 1, the neutral expressions in 374 videos of 97 models and one or two images of 6 ba...
Embodiment 2
[0070] Embodiment 2. Combining Gabor features and GentleSVM facial expression recognition
[0071] SVM is a general feed-forward neural network, which establishes a hyper-plane as a decision surface by minimizing structural risk, so that the margin between positive and negative examples is maximized. For expression recognition, the performance of SVM and Gentleboost is similar, and both have the highest performance in this field.
[0072] Gentleboost is used to select Gabor features, and SVM is trained on the new representation formed by feature selection to form the final classifier. In this study, this combination is called GentleSVM.
[0073] The data set used in the second embodiment is the same as that in the first embodiment. In order to test the generalization performance of the algorithm, a 10-fold cross-validation is used to evaluate the performance of the algorithm.
[0074] After the Gentleboost training is completed, the Gabor features used by the weak classifie...
Embodiment 2
[0078] Embodiment 2 compares the expression recognition performance of various GentleSVM algorithm combinations and the original SVM. The results are shown in Table 2 and Table 3.
[0079] Table 2 Expression recognition accuracy and training time of various GentleSVM algorithms
[0080]
[0081] Table 3 Recognition accuracy of various expressions
[0082]
[0083] As shown in Table 2, the accuracy rates of all GentleSVM algorithm combinations exceed the accuracy rates of the original SVM and the improved Gentleboost in Embodiment 1. In addition, all GentleSVM algorithm combinations completed 10 times of training within 20 seconds, which means that after completing Gentleboost training, the system can further improve performance with only a small cost of time. Among all GentleSVM algorithm combinations, the expression recognition accuracy of MI+DWT combination is the highest (92.66%). Combined with the results of Example 1, the above results show that the combination o...
PUM
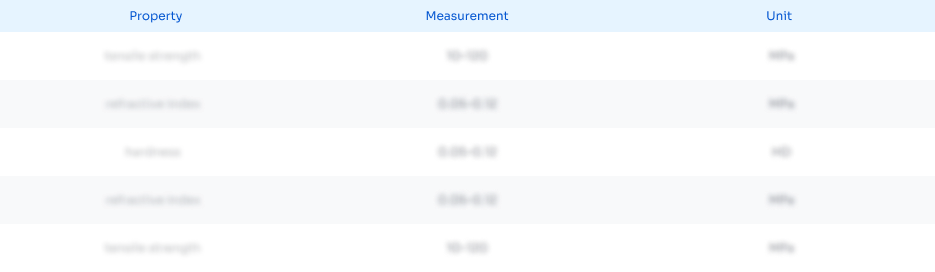
Abstract
Description
Claims
Application Information
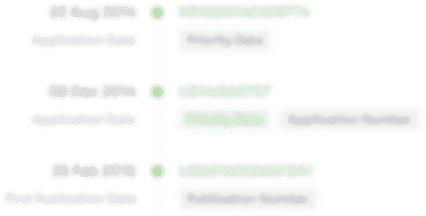
- R&D Engineer
- R&D Manager
- IP Professional
- Industry Leading Data Capabilities
- Powerful AI technology
- Patent DNA Extraction
Browse by: Latest US Patents, China's latest patents, Technical Efficacy Thesaurus, Application Domain, Technology Topic, Popular Technical Reports.
© 2024 PatSnap. All rights reserved.Legal|Privacy policy|Modern Slavery Act Transparency Statement|Sitemap|About US| Contact US: help@patsnap.com