Method for forecasting signal-to-noise ratio of mobile communication signals based on channel scene classification
A mobile communication and scene classification technology, applied in the field of signal-to-noise ratio prediction of mobile communication signals, can solve problems such as failure, performance impairment of adaptive communication systems, large deviation of signal-to-noise ratio prediction, etc., to achieve performance improvement, important application value and Scientific significance, effect of inhibiting damage
- Summary
- Abstract
- Description
- Claims
- Application Information
AI Technical Summary
Problems solved by technology
Method used
Image
Examples
Embodiment 1
[0069] Embodiment 1, signal-to-noise ratio prediction for channel slow-changing scenarios:
[0070] (1) Initialize the timer ;
[0071] (2) Set the measurement signal-to-noise ratio value (dB): u(1)=17.99; u(2)=18.28; u(3)=18.54; u(4)=18.88; u(5)=19.24; u(6)=19.47; u(7) =19.70; u(8)=20.02; u(9)=20.37; u(10)=20.68.
[0072] set up (dB), (dB); set two initial values of the system predictor: =15(dB), =10; Given = =4(dBw), parameter = =1;
[0073] The first 9 moments are all applicable to slow-changing scenes, from Start inputting sequentially, only inputting one signal-to-noise ratio value at a time, and use the following prediction formula:
[0074] >
[0075] >
[0076] >
[0077] will initial value and into the forecast formula >, >, >, after 9 iterations, we can get = Predicted value of sig...
Embodiment 2
[0081] Example 2, SNR prediction for scenarios with medium channel changes:
[0082] (1) Initialize the timer ;
[0083] (2) Set the measurement signal-to-noise ratio value (dB): u(1)=17.99; u(2)=18.28; u(3)=18.54; u(4)=18.88; u(5)=19.24; u(6)=19.47; u(7) =19.70; u(8)=20.02; u(9)=20.37; u(10)=23.68.
[0084] setting (dB), (dB); set two initial values of the system predictor: =15(dB), =10; Given = =4(dBw), parameter ==1;
[0085] The first 9 moments are all applicable to slow-changing scenes, from Start to input sequentially, only input one signal-to-noise ratio value at a time, and set the initial value and into the forecast formula >, >, >, after 9 iterations, we can get = Predicted signal-to-noise ratio value at time 10 It is 20.17 (dB).
[0086] =10 The absolute value of the deviation between the measured SNR value and the predicted SNR value ;because in range , the channel change scene at time = 10 is judged as a medium channel c...
Embodiment 3
[0093] Embodiment 3, the signal-to-noise ratio prediction of channel mutation scene:
[0094] (1) Initialize the timer ;
[0095] (2) Set the measurement signal-to-noise ratio value (dB): u(1)=17.99; u(2)=18.28; u(3)=18.54; u(4)=18.88; u(5)=19.24; u(6)=19.47; u(7) =19.70; u(8)=20.02; u(9)=20.37; u(10)=26.68; u(11)=21.14.
[0096] set up (dB), (dB); set two initial values of the system predictor: =15(dB), =10; Given = =4(dBw), parameter =1, c=0.95;
[0097] The first 9 moments are all applicable to slow-changing scenes, from Start to input sequentially, only input one signal-to-noise ratio value at a time, and set the initial value and into the forecast formula >, >, >, after 9 iterations, we can get = Predicted signal-to-noise ratio value at time 10 for (dB).
[0098] =10 The absolute value of the deviation between the measured SNR value and the predicted SNR value ;because in range in the =10 The channel change scene is ju...
PUM
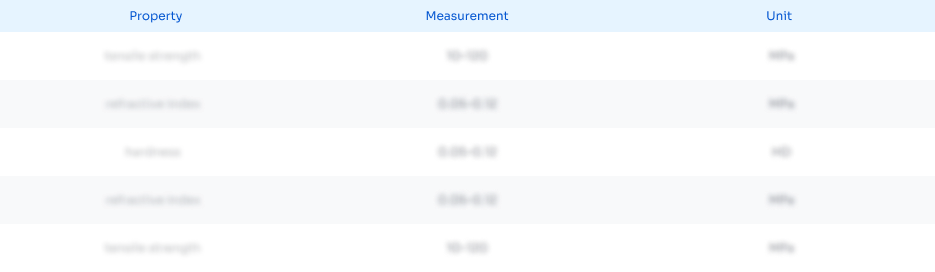
Abstract
Description
Claims
Application Information
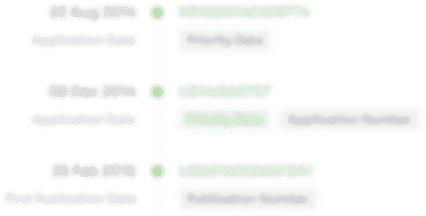
- R&D
- Intellectual Property
- Life Sciences
- Materials
- Tech Scout
- Unparalleled Data Quality
- Higher Quality Content
- 60% Fewer Hallucinations
Browse by: Latest US Patents, China's latest patents, Technical Efficacy Thesaurus, Application Domain, Technology Topic, Popular Technical Reports.
© 2025 PatSnap. All rights reserved.Legal|Privacy policy|Modern Slavery Act Transparency Statement|Sitemap|About US| Contact US: help@patsnap.com