Performance prediction method applicable to dynamic scheduling for semiconductor production line
A technology of dynamic scheduling and performance prediction, applied in prediction, data processing application, calculation, etc., can solve the problem that the learning speed cannot meet high real-time performance, and achieve the effect of convenient implementation, good real-time performance and high efficiency
- Summary
- Abstract
- Description
- Claims
- Application Information
AI Technical Summary
Problems solved by technology
Method used
Image
Examples
Embodiment Construction
[0025] To better explain the control method of the scheduling system of the present invention, please refer to figure 1 .
[0026] figure 1 Simplified model diagram for the semiconductor production line, classified by production process, there are three equipment groups, a total of five equipment, namely diffusion equipment group, ion implantation equipment group and lithography equipment group. The diffusion equipment group includes a first diffusion equipment Ma and a second diffusion equipment Mb; the ion implantation equipment group includes a first ion implantation equipment Mc and a second ion implantation equipment Md; and the lithography equipment group includes a lithography equipment Me. In front of each equipment group, a first buffer area B_Mab, a second buffer area B_Mcd, and a third buffer area B_Me are respectively set up to store information about workpieces that need to be processed. Through the above-mentioned equipment group, six processes can be realized, incl...
PUM
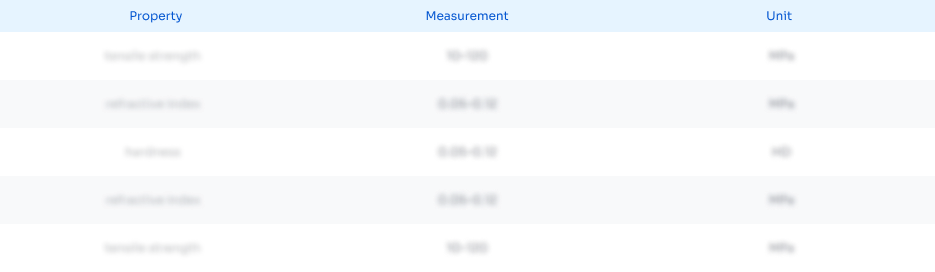
Abstract
Description
Claims
Application Information
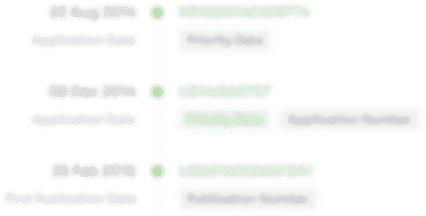
- R&D Engineer
- R&D Manager
- IP Professional
- Industry Leading Data Capabilities
- Powerful AI technology
- Patent DNA Extraction
Browse by: Latest US Patents, China's latest patents, Technical Efficacy Thesaurus, Application Domain, Technology Topic, Popular Technical Reports.
© 2024 PatSnap. All rights reserved.Legal|Privacy policy|Modern Slavery Act Transparency Statement|Sitemap|About US| Contact US: help@patsnap.com