Super-resolution image reconstruction method based on sparse multi-manifold embedment
A technology of super-resolution and image reconstruction, which is applied in the directions of image image conversion, image enhancement, image data processing, etc., and can solve problems such as inability to effectively represent image characteristics and affect the quality of image reconstruction
- Summary
- Abstract
- Description
- Claims
- Application Information
AI Technical Summary
Problems solved by technology
Method used
Image
Examples
Embodiment Construction
[0068] This invention proposes a super-resolution image reconstruction method based on sparse multi-manifold embedding, the key steps of which are the extraction of mid- and high-frequency feature vectors and the selection and embedding of high-frequency sparse neighbors. Before fuzzy downsampling, the rows and columns of the image should be adjusted first, so as to avoid the situation that the number of rows and columns is not uniform in the subsequent processing. The characteristics of this method are as follows: first construct a training feature library, extract mid- and high-frequency features from a large number of training images, obtain multiple training feature sets through clustering, and then extract mid-frequency features from low-resolution images that need to be restored, and then use mid-frequency feature matching , find the high-frequency neighbors from the nearest training feature set through sparse solving, and finally embed the high-frequency neighbors to obt...
PUM
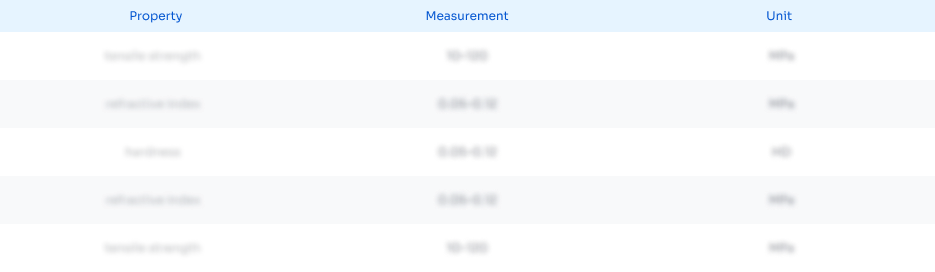
Abstract
Description
Claims
Application Information
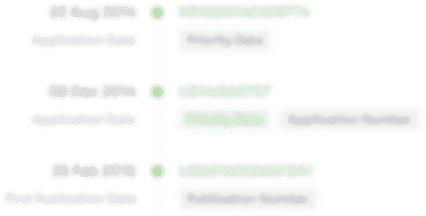
- R&D Engineer
- R&D Manager
- IP Professional
- Industry Leading Data Capabilities
- Powerful AI technology
- Patent DNA Extraction
Browse by: Latest US Patents, China's latest patents, Technical Efficacy Thesaurus, Application Domain, Technology Topic.
© 2024 PatSnap. All rights reserved.Legal|Privacy policy|Modern Slavery Act Transparency Statement|Sitemap