Regression-based active appearance model initialization method
A technology of active appearance model and initialization method, which is applied in the field of image analysis and can solve the problems of high positioning accuracy and large initialization error.
- Summary
- Abstract
- Description
- Claims
- Application Information
AI Technical Summary
Problems solved by technology
Method used
Image
Examples
Embodiment Construction
[0045] Combine below figure 1 The specific illustrations in the present invention are further elaborated.
[0046] refer to figure 1 The flowchart in the present invention realizes the regression-based active appearance model initialization method of the present invention. The algorithm first uses the kernel ridge regression algorithm (Kernel Ridge Regression, KRR) to establish the correspondence between the discrete local feature points and the structured calibration points in the training phase. Then in the test phase, assuming that the key feature points of the first frame are known, the double-threshold method is used to obtain the accurate local feature points corresponding to the previous frame and the current frame image, and then the discrete local feature points obtained by training are used to correspond to The mapping relationship between structured calibration points is used to extract the positioning information of the current frame's calibration points from the ...
PUM
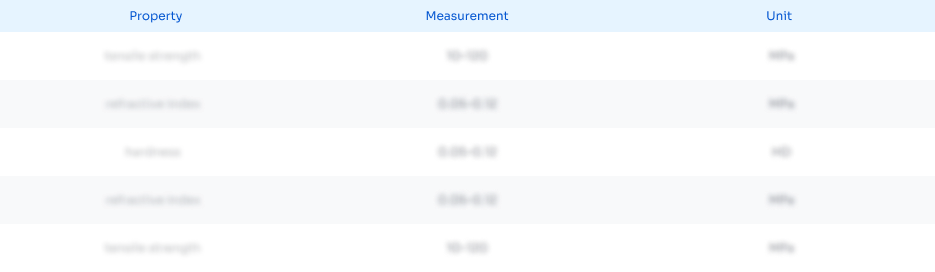
Abstract
Description
Claims
Application Information
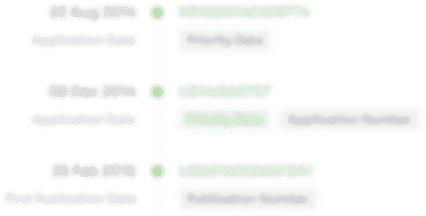
- Generate Ideas
- Intellectual Property
- Life Sciences
- Materials
- Tech Scout
- Unparalleled Data Quality
- Higher Quality Content
- 60% Fewer Hallucinations
Browse by: Latest US Patents, China's latest patents, Technical Efficacy Thesaurus, Application Domain, Technology Topic, Popular Technical Reports.
© 2025 PatSnap. All rights reserved.Legal|Privacy policy|Modern Slavery Act Transparency Statement|Sitemap|About US| Contact US: help@patsnap.com