Multi-level coal mine water inrush prediction method based on SaE-ELM (self-adaptive evolutionary extreme learning machine)
A prediction method and multi-level technology, applied in special data processing applications, instruments, electrical digital data processing, etc., can solve problems such as difficulty in building models and inconvenient preprocessing of different water inrush situations
- Summary
- Abstract
- Description
- Claims
- Application Information
AI Technical Summary
Problems solved by technology
Method used
Image
Examples
Embodiment 1
[0024] Example 1: To study the mechanism of coal mine water inrush, select the main controlling factors affecting coal mine water inrush and collect a large amount of historical data of coal mine water inrush as sample data. Each set of data includes each main controlling factor and the maximum water inrush. Normalize the data, divide the normalized data into training samples and test samples, and use SaE-ELM for training. The steps are as follows:
[0025] (1) N different training samples (x i ,t i ), where x i =[x i1 ,x i2 ,...,x in ]∈R n , t i =[t i1 ,t i2 ,...t im ]∈R m , the number of hidden layer nodes is L, and the activation function is g(x);
[0026] (2) Randomly generate NP original population vectors θ k,G , where k=1,2,…NP, each set of vectors contains all hidden layer parameters w j with b j , find the optimal θ through repeated mutation, crossover and selection operations during each training and testing process k,G ;
[0027] (3) Adjust the value...
PUM
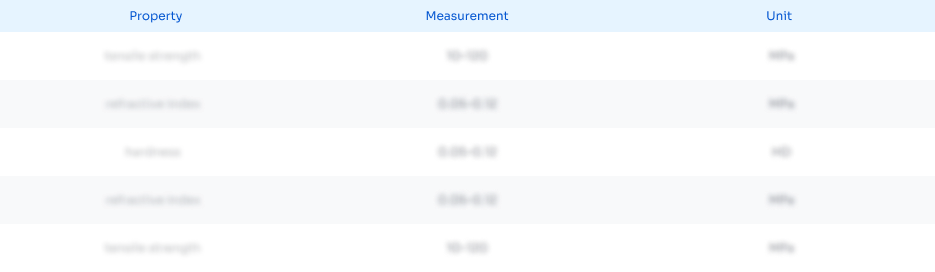
Abstract
Description
Claims
Application Information
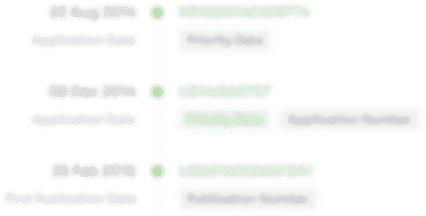
- R&D Engineer
- R&D Manager
- IP Professional
- Industry Leading Data Capabilities
- Powerful AI technology
- Patent DNA Extraction
Browse by: Latest US Patents, China's latest patents, Technical Efficacy Thesaurus, Application Domain, Technology Topic.
© 2024 PatSnap. All rights reserved.Legal|Privacy policy|Modern Slavery Act Transparency Statement|Sitemap