Local outlier detection method based on density clustering
A technology of outlier detection and density clustering, which is applied in the field of outlier detection, can solve the problems that the selection of parameter values is very sensitive, local outliers cannot be effectively detected, and it is difficult to determine
- Summary
- Abstract
- Description
- Claims
- Application Information
AI Technical Summary
Problems solved by technology
Method used
Image
Examples
Embodiment
[0059] Such as figure 1 , figure 2 As shown, a local outlier detection method based on density clustering described in this embodiment includes the following steps:
[0060] (a) Obtain the number of data families and cluster centers of the detected data set;
[0061] (b) by calculating the mean and standard deviation of the descriptive features of each data object in different data clusters;
[0062] (c) Use the 3sigma criterion to detect the outliers of each data cluster.
[0063] Step (a) is refined as follows:
[0064] (a1) Data set preprocessing: including data cleaning and data normalization. Data cleaning can manually delete noise data and data with missing values. To measure the influence of difference on data clustering and outlier detection process, it is necessary to normalize the data. Data normalization methods include maximum and minimum normalization, z-score normalization, and decimal scaling normalization.
[0065] (a2) Calculating the dissimilarity betwe...
PUM
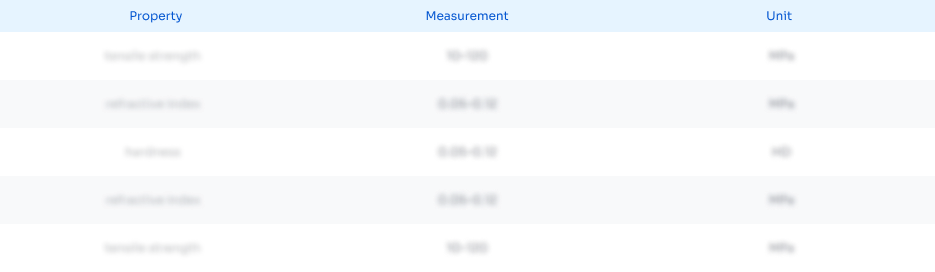
Abstract
Description
Claims
Application Information
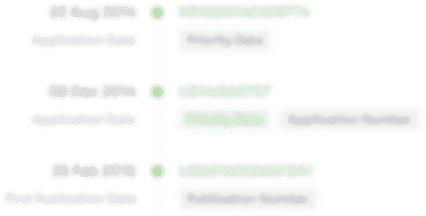
- Generate Ideas
- Intellectual Property
- Life Sciences
- Materials
- Tech Scout
- Unparalleled Data Quality
- Higher Quality Content
- 60% Fewer Hallucinations
Browse by: Latest US Patents, China's latest patents, Technical Efficacy Thesaurus, Application Domain, Technology Topic, Popular Technical Reports.
© 2025 PatSnap. All rights reserved.Legal|Privacy policy|Modern Slavery Act Transparency Statement|Sitemap|About US| Contact US: help@patsnap.com