Excavating diagnosis method of failure data based on bayesian network
A technology of Bayesian network and diagnostic method, which is applied in the field of fault data mining and diagnosis based on Bayesian network, which can solve the problems of destructiveness and equipment faults that do not exist independently, and achieve the effect of preventing overfitting
- Summary
- Abstract
- Description
- Claims
- Application Information
AI Technical Summary
Benefits of technology
Problems solved by technology
Method used
Image
Examples
Embodiment Construction
[0028] The following embodiments will further illustrate the present invention in conjunction with the accompanying drawings.
[0029] see figure 1 and 2 , the embodiment of the present invention includes the following steps:
[0030] 1) Take the listening signal and fault category as each input variable X of the Bayesian network, initialize each input variable X of the Bayesian network, including discretizing the non-discrete data to form a data table, and set the data table to contain n variables;
[0031] 2) Let the user input the number of tests m to determine the search density, and initialize the database D to save the Bayesian network and score obtained from a certain operation;
[0032] 3) Treat the n variables in step 1 as a Bayesian network N i n nodes, and randomly sort the n nodes and number them, such as 1, 2...n, check whether this random sorting already exists in the database D, if so, skip to step 11);
[0033] 4) Put the Bayesian network N i Initialized ...
PUM
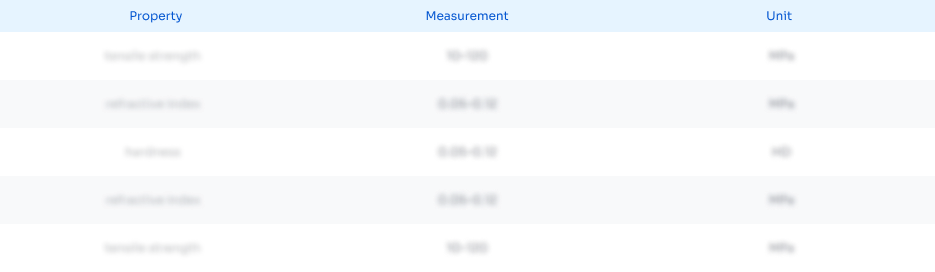
Abstract
Description
Claims
Application Information
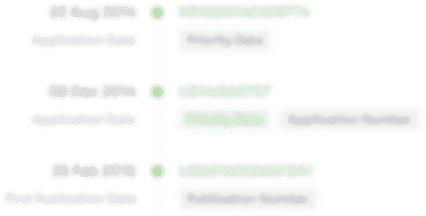
- R&D Engineer
- R&D Manager
- IP Professional
- Industry Leading Data Capabilities
- Powerful AI technology
- Patent DNA Extraction
Browse by: Latest US Patents, China's latest patents, Technical Efficacy Thesaurus, Application Domain, Technology Topic, Popular Technical Reports.
© 2024 PatSnap. All rights reserved.Legal|Privacy policy|Modern Slavery Act Transparency Statement|Sitemap|About US| Contact US: help@patsnap.com