Photovoltaic power generation output power tracking algorithm based on genetics algorithm improved RBF-BP neural network
A RBF-BP and neural network technology, applied in the field of photovoltaic power generation output power tracking algorithm, can solve problems such as incorrect training sample set misleading, and achieve strong self-adaptability, high prediction accuracy, and good generalization performance
- Summary
- Abstract
- Description
- Claims
- Application Information
AI Technical Summary
Problems solved by technology
Method used
Image
Examples
Embodiment Construction
[0055] like figure 1 As shown, the RBF-BP neural network has two hidden layers. The input data is trained as the input of the BP neural network subnet after being trained by the RBF neural network subnetwork. The network has the ability of error reverse learning. When the training result When the accuracy requirements are not met, the weights and thresholds of the neural network can be reversely modified until the training results meet the accuracy requirements.
[0056] like figure 2 As shown, the improved RBF-BP neural network algorithm based on the genetic algorithm is to confirm the output weight threshold length under the condition of confirming the network structure. The absolute value of the error between the output and the expected output is used as the fitness, and then the genetic algorithm is used to select, cross and mutate the data to find the individual corresponding to the optimal fitness, and then confirm the weight and threshold of the RBF-BP neural network ...
PUM
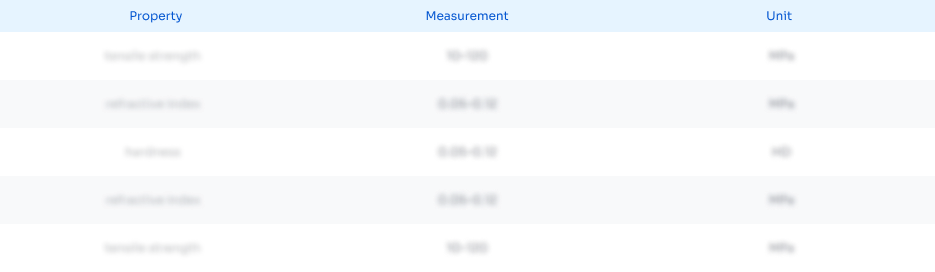
Abstract
Description
Claims
Application Information
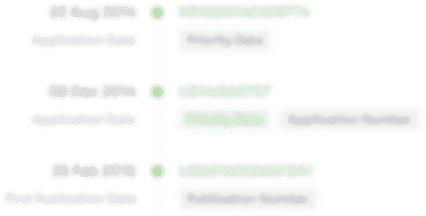
- R&D
- Intellectual Property
- Life Sciences
- Materials
- Tech Scout
- Unparalleled Data Quality
- Higher Quality Content
- 60% Fewer Hallucinations
Browse by: Latest US Patents, China's latest patents, Technical Efficacy Thesaurus, Application Domain, Technology Topic, Popular Technical Reports.
© 2025 PatSnap. All rights reserved.Legal|Privacy policy|Modern Slavery Act Transparency Statement|Sitemap|About US| Contact US: help@patsnap.com