Sparse self-adaptive semi-supervised manifold learning hyperspectral image classification method
An image classification, multi-manifold technology, applied in the field of hyperspectral data processing, can solve the problems of limiting algorithm identification ability, external learning, and not making full use of training sample category information.
- Summary
- Abstract
- Description
- Claims
- Application Information
AI Technical Summary
Problems solved by technology
Method used
Image
Examples
Embodiment
[0227] In order to verify the effectiveness of the method of the present invention, experiments are carried out through examples below, and under the same sample conditions, the method of the present invention is compared with other dimension reduction methods commonly used in the prior art. The dimensionality reduction methods used for comparison in this experiment are: Principal Component Analysis (PCA), Linear Discriminant Analysis (LDA), Neighborhood Preserving Embedding (NPE), Supervised NPE (Supervise NPE, SNPE), Locality Preserving Projection (LPP), Supervised LPP (Supervise LPP, SLPP), Marginal Fisher Analysis (MFA), Locality Fisher Discriminant Analysis (Locality Fisher Discriminant Analysis, LFDA), Maximum Margin Criterion (MMC), Sparsity Preserving Projections (SPP), Discriminative Learning based on Sparse Representation (Discriminative Learning by Sparse Representation, DLSP), Semi-supervised MMC (SSMMC), Semi-supervised MFA (Semi-supervised MFA, SSMFA), Semi-super...
PUM
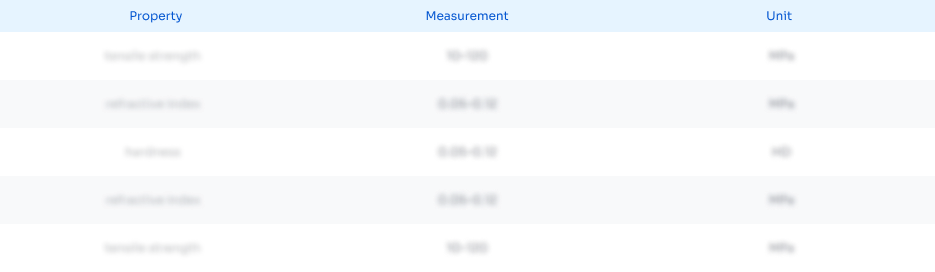
Abstract
Description
Claims
Application Information
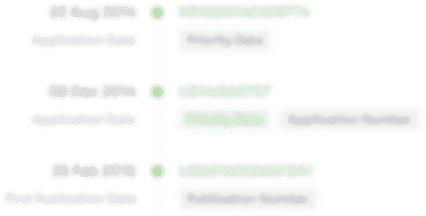
- R&D
- Intellectual Property
- Life Sciences
- Materials
- Tech Scout
- Unparalleled Data Quality
- Higher Quality Content
- 60% Fewer Hallucinations
Browse by: Latest US Patents, China's latest patents, Technical Efficacy Thesaurus, Application Domain, Technology Topic, Popular Technical Reports.
© 2025 PatSnap. All rights reserved.Legal|Privacy policy|Modern Slavery Act Transparency Statement|Sitemap|About US| Contact US: help@patsnap.com