Complex character recognition method based on deep learning
A text recognition and deep learning technology, applied in the field of image recognition, can solve a large number of problems such as human labeling and loss, and achieve the effect of solving information loss
- Summary
- Abstract
- Description
- Claims
- Application Information
AI Technical Summary
Problems solved by technology
Method used
Image
Examples
Embodiment 1
[0086] Such as Figure 8 As shown, first prepare a sample set of the same type as the picture to be recognized, for example, select 500 pictures with such Figure 9 The noise of the picture to be recognized and the sample pictures with similar fonts are manually marked, and 150 samples are selected as the development set, and the other 350 samples are used as the first training sample set; the strings in the pictures are segmented out , and split the string into sub-pictures that only include a single character, analyze the font of the picture to be recognized in the first training sample, and select the closest font: Times New Roman, then choose Times New Roman as the random sample generator Basic font library; if the characters contained in the picture with recognition are only numbers, you need to choose the Times New Roman number set as the basis for sample generation; according to the noise and distortion features contained in the artificially labeled samples (with Figu...
Embodiment 2
[0089] Such as Figure 11 As shown in the process of , when the character string has obvious characteristics of a certain language model, the recognition result of the deep neural network in the step (2-5) is optimized through the language model, and finally the language model optimized result is output Recognition results. For example, the target image to be recognized is Figure 12 As shown, the character string identified by the deep neural network is "Zhang San (the probability of "eating" is 50%, and the probability of "steam" is 50%) rice" wherein "Zhang San" and "rice" are identified Out of the probability of 100%, in this case according to the language structure model of the subject-verb-object in the speech model, the subject "Zhang San" and the object "rice" have been determined based on the middle character as the predicate verb "eat". The probability should be the largest, and "qi" is obviously impossible to appear in the position of the predicate verb as a noun,...
Embodiment 3
[0092] When the string to be recognized matches a specific language template, such as Figure 13 As shown, some language templates can be used to optimize the recognition results of the neural network. For example, the recognition results of picture 13 are "foolish", "valley", "moving" and "mountain"; the first, third and fourth characters are respectively recognized When the probability of being "foolish", "moving" and "mountain" is the highest (for example, 80%), the probability of recognizing the second character as "valley" is 60%. At this time, according to the fixed language template of the idiom, the The final result of the recognition is corrected to "Yugong Yishan"; this kind of recognition result is more in line with the correct language habits, and the recognition result is more accurate and reasonable.
PUM
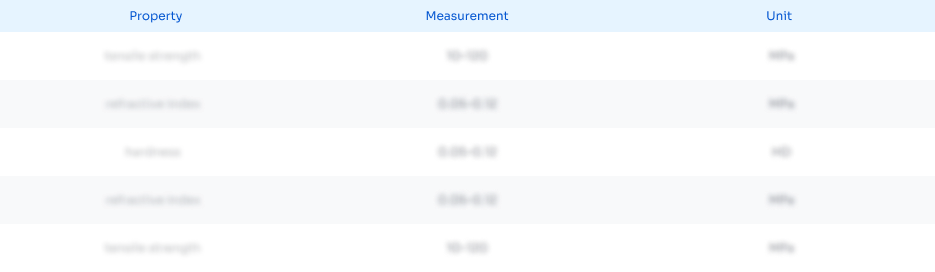
Abstract
Description
Claims
Application Information
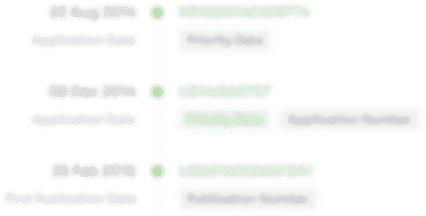
- R&D
- Intellectual Property
- Life Sciences
- Materials
- Tech Scout
- Unparalleled Data Quality
- Higher Quality Content
- 60% Fewer Hallucinations
Browse by: Latest US Patents, China's latest patents, Technical Efficacy Thesaurus, Application Domain, Technology Topic, Popular Technical Reports.
© 2025 PatSnap. All rights reserved.Legal|Privacy policy|Modern Slavery Act Transparency Statement|Sitemap|About US| Contact US: help@patsnap.com