Semi-supervised clustering method and semi-supervised clustering system based on nonnegative matrix factorization
A technique of non-negative matrix decomposition and semi-supervised clustering, which is applied in the field of semi-supervised clustering methods and systems, and can solve problems such as difficulty in obtaining information, failure to improve clustering performance, and ineffective use of the internal structure of original data.
- Summary
- Abstract
- Description
- Claims
- Application Information
AI Technical Summary
Problems solved by technology
Method used
Image
Examples
Embodiment Construction
[0054] The technical solutions in the embodiments of the present invention will be clearly and completely described below with reference to the accompanying drawings in the embodiments of the present invention. Obviously, the described embodiments are only a part of the embodiments of the present invention, but not all of the embodiments. Based on the embodiments of the present invention, all other embodiments obtained by those of ordinary skill in the art without creative efforts shall fall within the protection scope of the present invention.
[0055] like figure 1 As shown, a semi-supervised clustering method based on non-negative matrix decomposition disclosed in the present invention includes:
[0056] S101, performing a non-negative matrix decomposition projection on the original data matrix to obtain a low-dimensional approximate matrix of the original data with both neighborhood preservation and similarity preservation;
[0057] First, perform non-negative matrix deco...
PUM
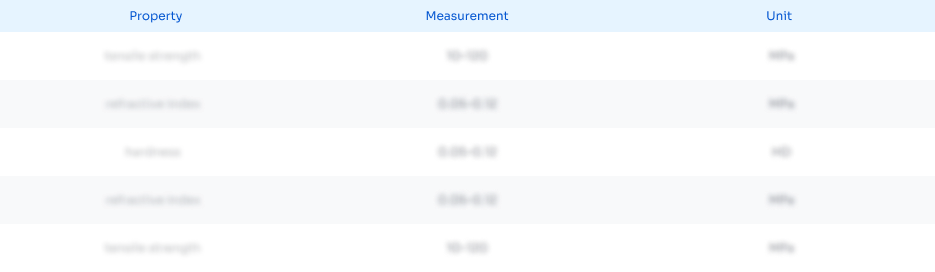
Abstract
Description
Claims
Application Information
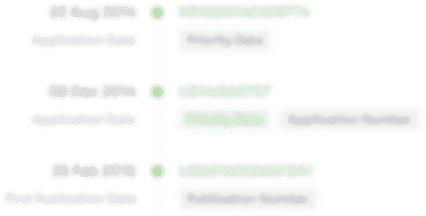
- R&D Engineer
- R&D Manager
- IP Professional
- Industry Leading Data Capabilities
- Powerful AI technology
- Patent DNA Extraction
Browse by: Latest US Patents, China's latest patents, Technical Efficacy Thesaurus, Application Domain, Technology Topic, Popular Technical Reports.
© 2024 PatSnap. All rights reserved.Legal|Privacy policy|Modern Slavery Act Transparency Statement|Sitemap|About US| Contact US: help@patsnap.com