Non-reference image quality objective evaluation method based on deep learning
A technology for objective quality evaluation and reference images, applied in image analysis, image communication, image data processing, etc., can solve the problems that classic deep learning models cannot directly apply image quality evaluation, are not accurate enough, and have high time complexity
- Summary
- Abstract
- Description
- Claims
- Application Information
AI Technical Summary
Problems solved by technology
Method used
Image
Examples
Embodiment Construction
[0027] The present invention will be further described in detail below in conjunction with the accompanying drawings and embodiments.
[0028] Since reference images cannot be obtained in many applications, the no-reference image quality assessment method is the most practical and challenging research topic, and the traditional no-reference image quality assessment has high computational complexity and time complexity, while the agreement between the objective quality of predictions and subjective perception is poor. The present invention extracts natural statistical features in the spatial domain by decomposing images, and the time complexity is very low. At the same time, multi-resolution pyramid and Gaussian difference decomposition can be used to perform multi-resolution analysis and multi-scale texture analysis on images, thereby extracting better natural statistics. feature; before the traditional shallow learning algorithm returns, the present invention adds a deep expr...
PUM
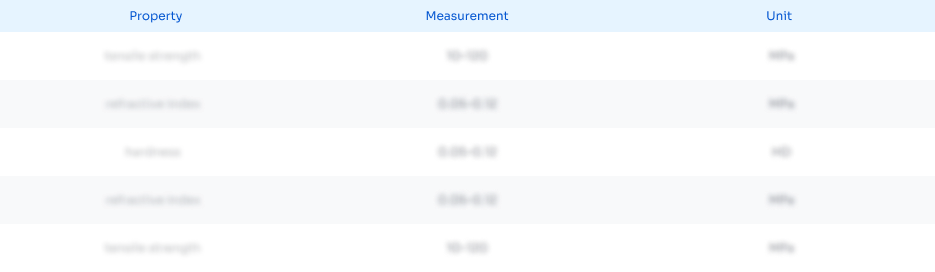
Abstract
Description
Claims
Application Information
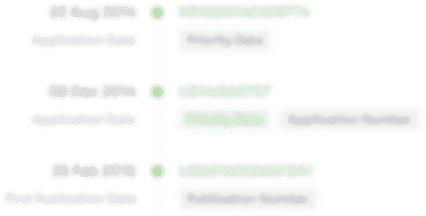
- R&D Engineer
- R&D Manager
- IP Professional
- Industry Leading Data Capabilities
- Powerful AI technology
- Patent DNA Extraction
Browse by: Latest US Patents, China's latest patents, Technical Efficacy Thesaurus, Application Domain, Technology Topic, Popular Technical Reports.
© 2024 PatSnap. All rights reserved.Legal|Privacy policy|Modern Slavery Act Transparency Statement|Sitemap|About US| Contact US: help@patsnap.com