A Fully Supervised Classification Method of Remote Sensing Images Based on SIFT Training Sample Extraction
A technology of training samples and classification methods, which is applied in the directions of instruments, calculations, character and pattern recognition, etc., can solve the problems of high algorithm complexity, difficulty in obtaining effective labeled samples, high dependence on training samples, etc., and achieve the effect of good representation ability
- Summary
- Abstract
- Description
- Claims
- Application Information
AI Technical Summary
Problems solved by technology
Method used
Image
Examples
Embodiment Construction
[0054] The present invention will be described in detail below in conjunction with the accompanying drawings and specific embodiments.
[0055] like figure 1 As shown, the specific implementation steps of the remote sensing image full-supervised classification method extracted based on SIFT training samples of the present invention are as follows:
[0056] (1) The process of normalizing the magnitude data of the initial image is specifically as follows:
[0057] The normalization formula is:
[0058]
[0059] Among them, R is the initial image, R Norm is the normalized representation of the corresponding amplitude data; select R Norm The data whose amplitude value is in the range of (0, eps) is equal to 99.8% is used as the experimental data, and the data larger than eps is set as eps.
[0060] (2) Using SIFT and Laplacian detail enhancement operator to extract the key points in the normalized image as candidate samples, the process is as follows: first construct the no...
PUM
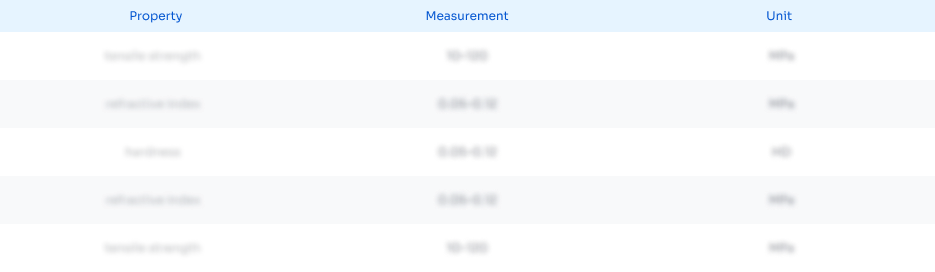
Abstract
Description
Claims
Application Information
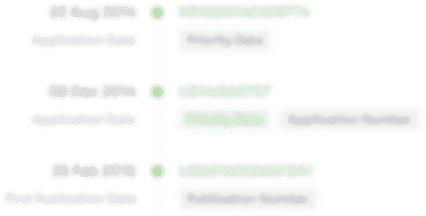
- R&D Engineer
- R&D Manager
- IP Professional
- Industry Leading Data Capabilities
- Powerful AI technology
- Patent DNA Extraction
Browse by: Latest US Patents, China's latest patents, Technical Efficacy Thesaurus, Application Domain, Technology Topic, Popular Technical Reports.
© 2024 PatSnap. All rights reserved.Legal|Privacy policy|Modern Slavery Act Transparency Statement|Sitemap|About US| Contact US: help@patsnap.com