Behavior Recognition Based on 3D Point Cloud and Key Skeletal Nodes
A 3D and node technology, which is applied in the field of behavior recognition based on 3D point cloud and key skeletal nodes, can solve the problems of ignoring geometry and topology, low recognition rate of complex behavior, difficult trade-off between real-time processing and high-precision recognition, etc. Achieve the effect of improving the accuracy of behavior recognition and improving the accuracy of recognition
- Summary
- Abstract
- Description
- Claims
- Application Information
AI Technical Summary
Problems solved by technology
Method used
Image
Examples
Embodiment 1
[0025]Action Recognition on the MSR-Action 3D Dataset. The MSR-Action 3D data set contains 20 behaviors, namely: high arm swing, cross arm swing, hammering, grasping, forward boxing, high throwing, drawing X, drawing hook, drawing circle, clapping hands, swinging with both hands , Side boxing, bending, forward kicking, side kicking, jogging, tennis swing, tennis serve, golfing, picking up, and throwing, each act is performed 2 or 3 times by 10 people. The actors collected in this database are in a fixed position, and most of the behaviors mainly involve the movement of the upper body of the actor. First, we directly extract the 3D point cloud sequence from the depth sequence, and divide the 3D point cloud sequence into non-overlapping (24×32×18) and (24×32×12) along the X, Y, and T directions respectively. spatio-temporal units; our method is then tested using cross-validation, i.e. five subjects for training and the remaining five for testing, exhaustively 252 times. Table ...
Embodiment 2
[0027] Action Recognition on the MSR Daily Activity 3D Dataset. The data set contains 16 behaviors, consisting of 10 behavior subjects, each behavior subject performs the behavior 2 times, one standing, one sitting, a total of 320 behavior videos. The 16 behaviors are: drinking, eating, reading, talking on the phone, writing, sitting, using a notebook, vacuum cleaning, laughing, throwing paper, playing games, lying on the sofa, walking, playing guitar, standing, and sitting. The experimental setup is the same as above, this database is extremely challenging, not only contains intra-class variation, but also involves human-object interaction behavior. Table 2 is a comparison of the recognition rates of different methods on this database. It can be seen from the table that our method has achieved an accuracy rate of 98.1%, and the average accuracy rate has reached 94.0±5.68%, which is an excellent experimental result.
Embodiment 3
[0029] Action Recognition on the MSR Action Pairs 3D Dataset. This data set is a data set of behavior pairs, including 12 behaviors and 6 groups of behavior pairs, namely: pick up a box, put down a box, lift a box, place a box, push a chair, pull a chair , put on a hat, take off a hat, carry a backpack, take off a backpack, stick a poster, pull a poster. In this database, there are similar motion and shape cues between each behavior pair, but their temporal correlation is opposite. The experimental settings are the same as above. Table 3 is the comparison of all existing popular methods on this database. Our method has achieved a recognition rate of 97.2%.
[0030] Table 1: Performance of existing methods on the MSR Action 3D dataset. Mean±STD is calculated from 252 cycles. The 5 / 5 column means that {1,3,5,7,9} of the agents are used for training and the rest are used for testing.
[0031]
[0032] Table 2: Comparison of MSR Daily Activity recognition rates. Mean±STD i...
PUM
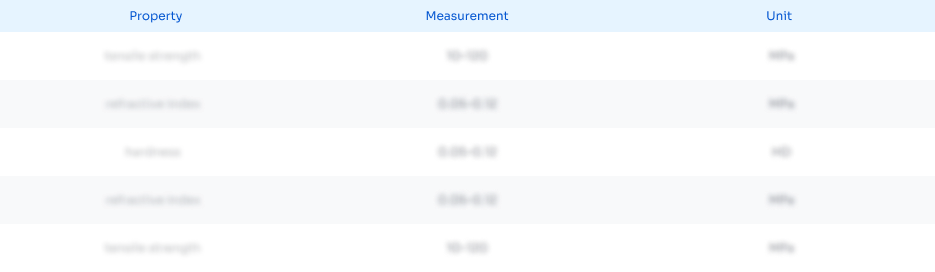
Abstract
Description
Claims
Application Information
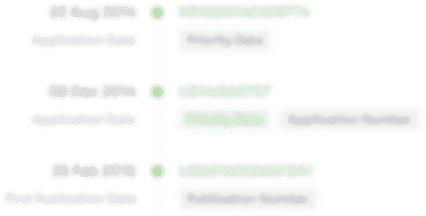
- R&D Engineer
- R&D Manager
- IP Professional
- Industry Leading Data Capabilities
- Powerful AI technology
- Patent DNA Extraction
Browse by: Latest US Patents, China's latest patents, Technical Efficacy Thesaurus, Application Domain, Technology Topic, Popular Technical Reports.
© 2024 PatSnap. All rights reserved.Legal|Privacy policy|Modern Slavery Act Transparency Statement|Sitemap|About US| Contact US: help@patsnap.com