System and method for establishing target division remote damage assessment of different vehicle types based on artificial intelligence semi-supervised learning BIRCH method
A semi-supervised learning and artificial intelligence technology, applied in the field of vehicle loss assessment, to achieve the effect of improving accuracy
- Summary
- Abstract
- Description
- Claims
- Application Information
AI Technical Summary
Problems solved by technology
Method used
Image
Examples
Embodiment 1
[0025] A BIRCH method based on artificial intelligence semi-supervised learning to establish a remote damage assessment system for different vehicle models, including:
[0026] The model selection subsystem selects the model data corresponding to the vehicle as the total data set;
[0027] The data classification subsystem reads CAE simulation data and real vehicle data, and classifies the data accordingly;
[0028] The collision detection subsystem judges whether the vehicle collides during driving; the collision detection subsystem learns the collision training data to generate a collision model, and the collision model is established using the intelligent semi-supervised learning BIRCH method;
[0029] The working condition detection subsystem judges all the working condition information of the collision; the working condition detection subsystem learns the working condition training data to generate a working condition model, and the working condition model is established ...
Embodiment 2
[0054] An artificial intelligence semi-supervised learning BIRCH method to establish a remote damage assessment method for different vehicle types according to the target, comprising the following steps:
[0055] Step 1. Select the model data corresponding to the vehicle as the total data set;
[0056] Step 2. Read the CAE simulation data and real vehicle data, and classify the data accordingly;
[0057] Step 3. Judging whether the vehicle collides during driving; the collision detection subsystem learns the collision training data so as to generate a collision model, and the collision model is set up using an intelligent semi-supervised learning BIRCH method;
[0058] Step 4. Judging all working condition information that collision occurs; The working condition detection subsystem learns working condition training data so as to generate a working condition model, and the working condition model is set up using an intelligent semi-supervised learning BIRCH method;
[0059] St...
Embodiment 3
[0091] Example 3: Have the technical scheme identical with embodiment 1 or 2, more specifically:
[0092] The overall data set in the above scheme: all are CAE simulation data and sports car data; it is divided into three parts as follows
[0093] 1. Training data set: it is used to train the model or determine the model parameters (CAE simulation data and sports car data).
[0094] 2. Verification data set: It is used for model selection, that is, for final optimization and determination of the model (CAE simulation data and sports car data).
[0095] 3. Test data set: it is purely to test the generalization ability of the trained model. (CAE simulation data and sports car data).
PUM
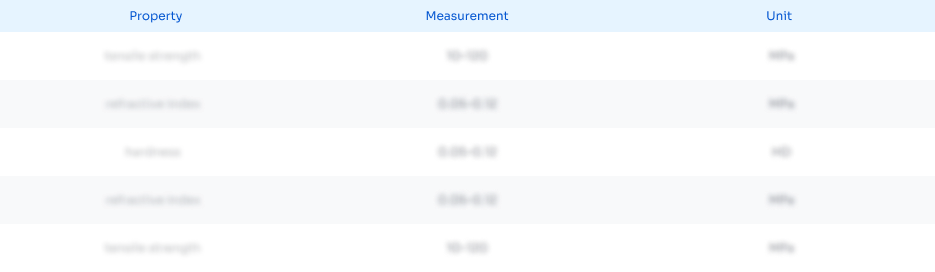
Abstract
Description
Claims
Application Information
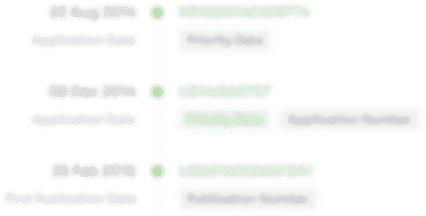
- R&D
- Intellectual Property
- Life Sciences
- Materials
- Tech Scout
- Unparalleled Data Quality
- Higher Quality Content
- 60% Fewer Hallucinations
Browse by: Latest US Patents, China's latest patents, Technical Efficacy Thesaurus, Application Domain, Technology Topic, Popular Technical Reports.
© 2025 PatSnap. All rights reserved.Legal|Privacy policy|Modern Slavery Act Transparency Statement|Sitemap|About US| Contact US: help@patsnap.com