Deep strategy learning method facing complex missions in large-scale environment
A technology for policy learning and complex tasks, applied in the field of policy search reinforcement learning algorithms for continuous state action spaces, can solve problems such as the limitations of reinforcement learning, and achieve the effects of strong generalization ability, improved automation, and good technical support
- Summary
- Abstract
- Description
- Claims
- Application Information
AI Technical Summary
Problems solved by technology
Method used
Image
Examples
Embodiment Construction
[0035] The present invention will be further described in detail below in conjunction with the accompanying drawings and through specific embodiments. The following embodiments are only descriptive, not restrictive, and cannot limit the protection scope of the present invention.
[0036] In the implementation process of the reinforcement learning scheme for complex tasks in a large-scale environment described in the present invention, the interaction process between the agent and the environment is modeled as a Markov decision process (MDP), which can use a quadruple to represent (S, A, P T , P I , r, γ): where S represents the continuous state space, A represents the continuous action space, and P T (s t+1 |s t , a t ) means that in the current state s t take action a t then transition to the next state s t+1 The state transition probability density function of P I (s 1 ) is the initial state probability density function of the agent, r(s t , a t ,s t+1 ) represen...
PUM
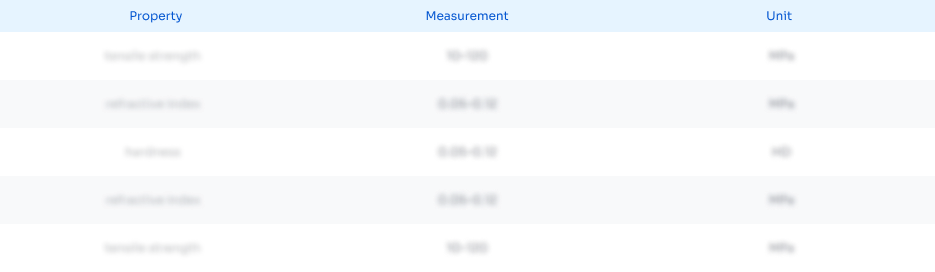
Abstract
Description
Claims
Application Information
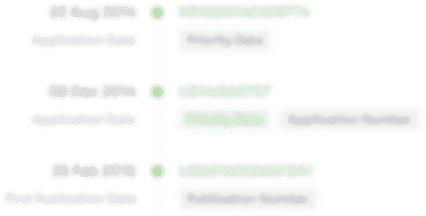
- R&D
- Intellectual Property
- Life Sciences
- Materials
- Tech Scout
- Unparalleled Data Quality
- Higher Quality Content
- 60% Fewer Hallucinations
Browse by: Latest US Patents, China's latest patents, Technical Efficacy Thesaurus, Application Domain, Technology Topic, Popular Technical Reports.
© 2025 PatSnap. All rights reserved.Legal|Privacy policy|Modern Slavery Act Transparency Statement|Sitemap|About US| Contact US: help@patsnap.com