Horizontal Decision Tree Learning from Very High Rate Data Streams
A technique of decision tree, data processing system, applied in the field of improved data processing device
- Summary
- Abstract
- Description
- Claims
- Application Information
AI Technical Summary
Problems solved by technology
Method used
Image
Examples
Embodiment Construction
[0023] Real-world applications of big data stream processing present several challenges. The data arrival rate is high. For example, in a small-scale connected vehicle platform, a Global Positioning System (GPS) application considers one million GPS data instances per second. Also, the number of data attributes (feature size) can be large. For example, real-time text analysis considers ten thousand or more attributes. With data arriving twenty-four hours a day and seven days a week, the amount of data to consider can be unlimited.
[0024] The illustrative embodiments provide mechanisms that enable horizontal decision trees to learn from very high rates of data streams. In some applications, such as in connected cars or vehicle-to-vehicle communication scenarios, the number of attributes is small, but the data rate is very high. The mechanisms of the illustrative embodiments horizontally parallelize the most computationally intensive part of horizontal decision tree learni...
PUM
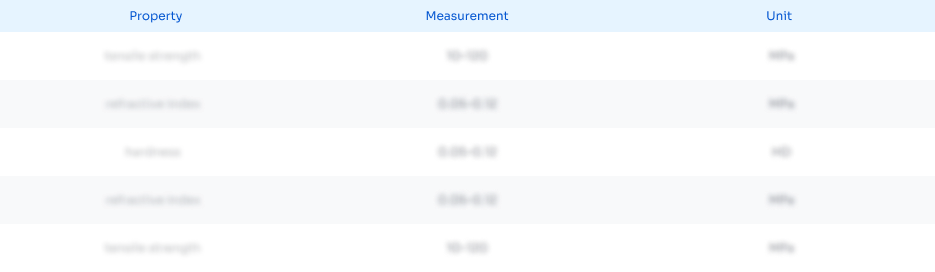
Abstract
Description
Claims
Application Information
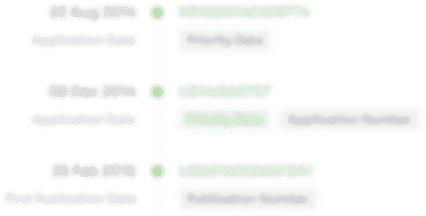
- Generate Ideas
- Intellectual Property
- Life Sciences
- Materials
- Tech Scout
- Unparalleled Data Quality
- Higher Quality Content
- 60% Fewer Hallucinations
Browse by: Latest US Patents, China's latest patents, Technical Efficacy Thesaurus, Application Domain, Technology Topic, Popular Technical Reports.
© 2025 PatSnap. All rights reserved.Legal|Privacy policy|Modern Slavery Act Transparency Statement|Sitemap|About US| Contact US: help@patsnap.com