Deep learning model based on multi-scale network and application in brain state monitoring
A deep learning, multi-scale technology, applied in the direction of biological neural network models, applications, neural learning methods, etc.
- Summary
- Abstract
- Description
- Claims
- Application Information
AI Technical Summary
Problems solved by technology
Method used
Image
Examples
Embodiment Construction
[0057] The multi-scale network-based deep learning model of the present invention and its application in brain state monitoring will be described in detail below in conjunction with the embodiments and drawings.
[0058] The multi-scale network-based deep learning model of the present invention and its application in brain state monitoring calculate the cross-recursion rate between each pair of signals on multiple scales through the multi-channel EEG signals measured by smart wearable devices, so as to The cross recurrence rate determines the edge weight of the recurrent network, and the signal of each channel is used as the node of the network to establish a multi-scale weighted recurrent network. Convert a multiscale weighted recurrent network to an unweighted recurrent network by picking a threshold. Extract a large number of indicators of the network, use them as the input of the deep learning model, and build a deep learning model based on a multi-scale recurrent network ...
PUM
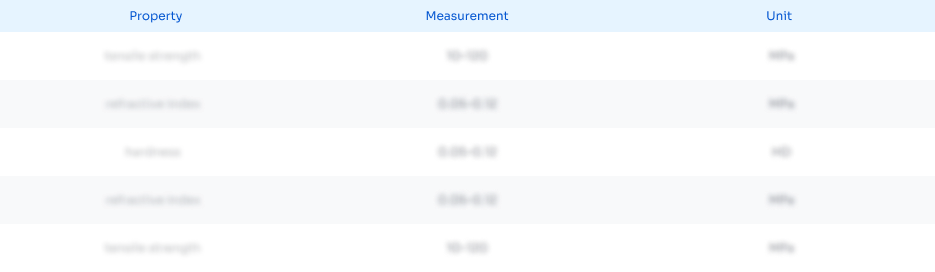
Abstract
Description
Claims
Application Information
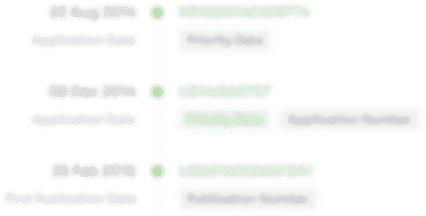
- R&D
- Intellectual Property
- Life Sciences
- Materials
- Tech Scout
- Unparalleled Data Quality
- Higher Quality Content
- 60% Fewer Hallucinations
Browse by: Latest US Patents, China's latest patents, Technical Efficacy Thesaurus, Application Domain, Technology Topic, Popular Technical Reports.
© 2025 PatSnap. All rights reserved.Legal|Privacy policy|Modern Slavery Act Transparency Statement|Sitemap|About US| Contact US: help@patsnap.com