Analysis method and application of electroencephalogram (EEG) signals based on complex network
A technology of EEG signals and complex networks, applied in applications, medical science, sensors, etc., can solve the problems of patients' physical and mental and intellectual influences, and achieve high accuracy results
- Summary
- Abstract
- Description
- Claims
- Application Information
AI Technical Summary
Problems solved by technology
Method used
Image
Examples
example 1
[0067] The EEG data sets of five epilepsy patients during the seizure period and the EEG data sets of five healthy people in the relaxed state were collected respectively. The EEG signals of each subject were multi-channel EEG signals with 20 electrodes. It adopts 10-20 international standard placement, the sampling frequency is 173.61Hz, and the sampling time is 23.6 seconds. After preprocessing the collected raw EEG data, the denoised EEG data can be obtained. Construct the multi-scale horizontal finite traversing visual complex network of each preprocessed EEG signal, where the finite traversing visual distance L=1, and calculate the average clustering coefficient and aggregation of nodes in the horizontal finite traversing visual complex network at different scales. Coefficient entropy value, based on leave-one-out cross-validation and ten-fold cross-validation, the method of the present invention can achieve accurate classification of EEG data in epilepsy and healthy brai...
example 2
[0069] Collect the EEG signals of the deep sleep stage and the rapid eye movement stage of 25 adults with sleep disorders, respectively. For the collected data, construct a complex network of multi-scale horizontal limited traversal visualization of each EEG signal. , where the limited crossing sight distance L=1, calculate the average aggregation coefficient and aggregation coefficient entropy value of the nodes of the complex network of horizontal limited crossing visible view at different scales, based on the leave-one-out cross-validation, the method of the present invention can realize the different sleep stages. The accurate classification of the EEG data in the brain state has an accuracy rate of 97%. Based on the ten-fold cross-validation, the method of the invention can also realize the accurate classification of the EEG data in the brain state of different sleep stages, and the accuracy rate can reach 97.33%. Therefore, the method of the present invention can effectiv...
PUM
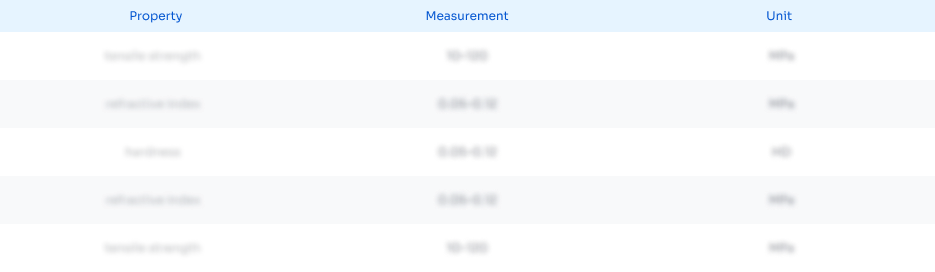
Abstract
Description
Claims
Application Information
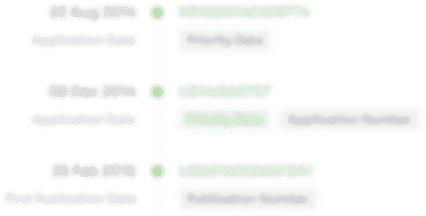
- Generate Ideas
- Intellectual Property
- Life Sciences
- Materials
- Tech Scout
- Unparalleled Data Quality
- Higher Quality Content
- 60% Fewer Hallucinations
Browse by: Latest US Patents, China's latest patents, Technical Efficacy Thesaurus, Application Domain, Technology Topic, Popular Technical Reports.
© 2025 PatSnap. All rights reserved.Legal|Privacy policy|Modern Slavery Act Transparency Statement|Sitemap|About US| Contact US: help@patsnap.com