Weak signal enhancement detection method based on complementary stochastic resonance filter
A stochastic resonance and detection method technology, which is applied in the testing of machines/structural components, mechanical components, mechanical bearings, etc., can solve the problems affecting the accuracy of fault diagnosis and unsatisfactory filtering effect, so as to improve and enhance the detection effect , to achieve the effect of adaptive enhancement and accuracy improvement
- Summary
- Abstract
- Description
- Claims
- Application Information
AI Technical Summary
Problems solved by technology
Method used
Image
Examples
Embodiment 1
[0035] In order to verify the weak signal enhanced detection performance of the complementary stochastic resonance filter proposed by the present invention, at first the noise-containing sinusoidal signal is analyzed, and the parameters of the noise-containing sinusoidal signal are set to: A 1 =A 2 =1,f 0 =100Hz, Its waveform and spectrum are as figure 2 shown. It can be seen from the time domain waveform that the sinusoidal signal is completely submerged by noise. It can be seen from the frequency spectrum that the driving frequency f 0 , but the noise interference is very obvious. Bundle figure 2 The noisy signal of is split in half into two sub-signals S 1 (t) and S 2 (t) and input to the two input ends of the complementary stochastic resonance filter, in the optimal parameter δ 1 =-1.0e6,δ 2 =-1.0e6,S R1 =1.0e4,S R2 =1.0e6, T R1 =1.0e-1, T R2 =1.1e-1, the optimal output of the complementary stochastic resonance filter is as follows image 3 shown. It can...
Embodiment 2
[0037] In order to further verify the superiority of the present invention in weak signal enhanced detection, the inner ring signal of the bearing fault is analyzed. The model of the inner ring fault bearing is shown in Table 1:
[0038] Table 1 Inner ring fault bearing parameters and fault information
[0039]
[0040] When the inner ring of the bearing has a partial fault, the moving rollers will pass through the faulty part of the inner ring in turn and collide to generate shock vibration, which further causes machine resonance. Therefore, the bearing fault signal is composed of a series of attenuated shock signals modulated by resonance frequency. On the other hand, at a certain speed, the time interval between two adjacent impact signals (the reciprocal of which is the fault characteristic frequency) can reflect different fault types of bearings. Since the fault signal is an amplitude modulated signal, the resonance demodulation technique is often used to demodulate t...
Embodiment 3
[0042] In order to further verify the superiority and effectiveness of the method proposed by the present invention, the bearing outer ring fault signal is analyzed, and the bearing model is shown in Table 2:
[0043] Table 2 Outer ring fault bearing parameters and fault information
[0044]
[0045] Analysis results such as Figure 6 with Figure 7 shown, from Figure 6 It can be seen from the figure that the fault characteristic frequency f of the outer ring of the bearing is o Ambient noise is very noticeable, interfering with accurate diagnosis of bearing faults. The resulting output of the complementary stochastic resonance filter is as follows Figure 7 As shown, the optimal parameter is: δ 1 =-7.9e4,δ 2 =1.0e9,S R1 =4.0e4,S R2 =1.0e7,T R1 =1.0, T R2 = 1.0. The energy of high frequency and low frequency noise in the frequency spectrum is reduced, and the fault characteristic frequency f o is effectively enhanced, so that it can be clearly judged that there...
PUM
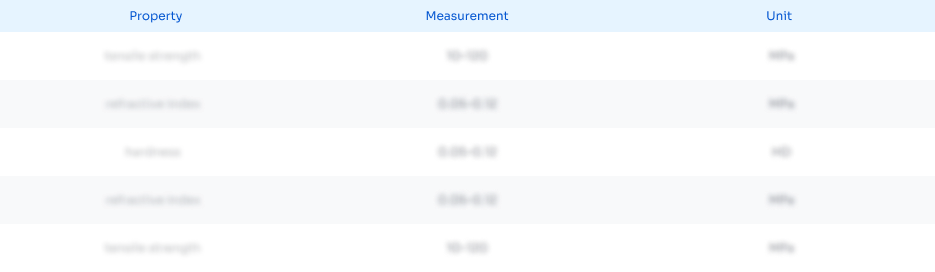
Abstract
Description
Claims
Application Information
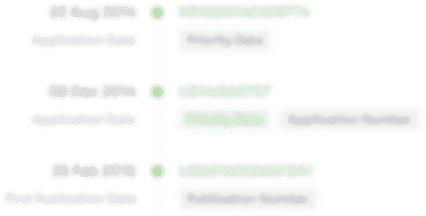
- Generate Ideas
- Intellectual Property
- Life Sciences
- Materials
- Tech Scout
- Unparalleled Data Quality
- Higher Quality Content
- 60% Fewer Hallucinations
Browse by: Latest US Patents, China's latest patents, Technical Efficacy Thesaurus, Application Domain, Technology Topic, Popular Technical Reports.
© 2025 PatSnap. All rights reserved.Legal|Privacy policy|Modern Slavery Act Transparency Statement|Sitemap|About US| Contact US: help@patsnap.com