Cluster-based multi-label imbalance biomedical data classification method
A data classification and biomedical technology, applied in the multi-label field, can solve problems such as multi-label imbalanced biomedical data classification methods, multi-label imbalanced biomedical data classification performance errors, etc., to improve reliability and reduce noise data The effect of probability
- Summary
- Abstract
- Description
- Claims
- Application Information
AI Technical Summary
Problems solved by technology
Method used
Image
Examples
Embodiment Construction
[0020] The preferred embodiments of the present invention will be described in detail below with reference to the accompanying drawings.
[0021] refer to figure 1 , figure 1 A flow chart of a clustering-based multi-label imbalanced biomedical data classification method provided in this embodiment, specifically including:
[0022] 101: Define an association matrix for biomedical data according to feature similarity and label association.
[0023] A new clustering method is defined in the unbalanced multi-label data space. This clustering method not only considers the similarity between features, but also considers the association of multi-label space when clustering biomedical sample data. , and then establishes an association to define an association matrix through the similarity between features and the association of the multi-label space.
[0024] The correlation matrix refers to the correlation matrix obtained by comprehensively considering feature similarity and label...
PUM
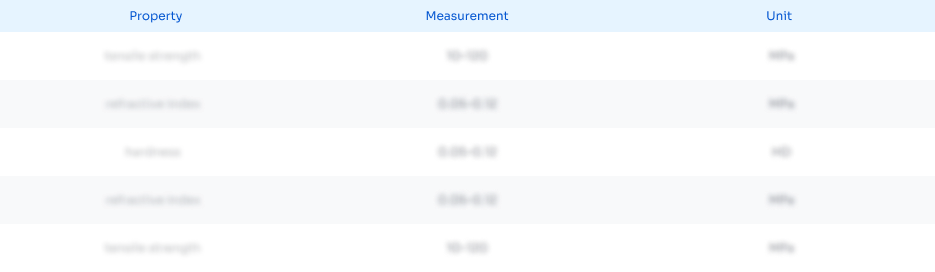
Abstract
Description
Claims
Application Information
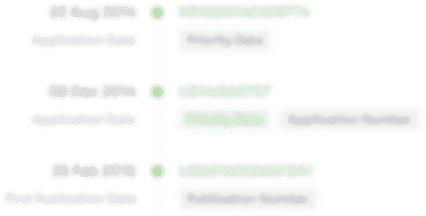
- R&D Engineer
- R&D Manager
- IP Professional
- Industry Leading Data Capabilities
- Powerful AI technology
- Patent DNA Extraction
Browse by: Latest US Patents, China's latest patents, Technical Efficacy Thesaurus, Application Domain, Technology Topic, Popular Technical Reports.
© 2024 PatSnap. All rights reserved.Legal|Privacy policy|Modern Slavery Act Transparency Statement|Sitemap|About US| Contact US: help@patsnap.com