Intelligent power grid short-term load predication method based on improved RBF neural network
A short-term load forecasting, smart grid technology, applied in neural learning methods, biological neural network models, forecasting, etc., can solve the problems of low accuracy, complex methods, and many dimensions of smart grid load forecasting
- Summary
- Abstract
- Description
- Claims
- Application Information
AI Technical Summary
Problems solved by technology
Method used
Image
Examples
Embodiment 1
[0043] This embodiment proposes a smart grid short-term load forecasting method based on an improved RBF neural network. This method uses the PCA-WFCM clustering algorithm to determine the RBF basis function center c i , using the gradient descent method to determine the connection weights between the hidden layer and the output layer of the RBF neural network. The following is a detailed description of the smart grid short-term load forecasting method based on the improved RBF neural network:
[0044] First establish sample data X={x 1 ,x 2 ,...,x N},x j For each sample point, x j ={x j1 ,x j2 ,...,x js}, j=1,2,...N, there are N sample points in total, x j Indicates that each sample point contains s attributes. In cluster analysis, the sample data X is divided into K categories, and the range of K is 2≤K≤N; the cluster center V={v 1 ,v 2 ,...,v k}, a total of K cluster centers.
[0045] Radial basis function (RBF) neural network is a feed-forward neural network b...
Embodiment 2
[0079] According to the idea in the first embodiment, the neural network prediction in this embodiment needs to determine the input and output of the neural network and the number of hidden layer nodes in advance. The network input is determined by a series of parameters that affect the predicted value. Because the smart grid user load curve has good periodic characteristics, the influence of the load value at a certain moment can consider the daily cycle characteristics and weekly cycle characteristics, that is, the load value at the same time of the day before the forecast time and the load value at the same time of the previous week can be selected . Specifically predict the number of neurons in the input layer N Ⅰ =9, including 8 load points: load value L(t-1) at the moment before the forecast point, load value L(t-2) at two moments before the forecast point, load value L(t-48) at the same forecast point the day before , Load value L at the moment before the same predict...
PUM
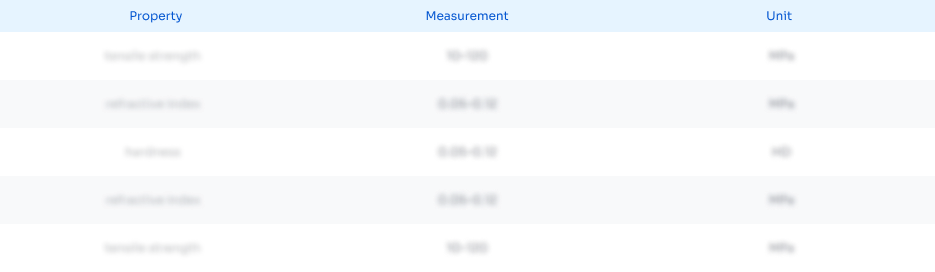
Abstract
Description
Claims
Application Information
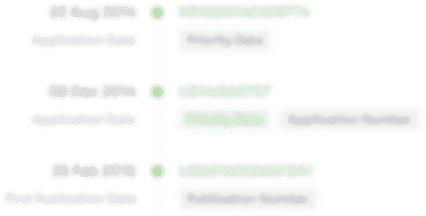
- R&D Engineer
- R&D Manager
- IP Professional
- Industry Leading Data Capabilities
- Powerful AI technology
- Patent DNA Extraction
Browse by: Latest US Patents, China's latest patents, Technical Efficacy Thesaurus, Application Domain, Technology Topic, Popular Technical Reports.
© 2024 PatSnap. All rights reserved.Legal|Privacy policy|Modern Slavery Act Transparency Statement|Sitemap|About US| Contact US: help@patsnap.com