RGB-D image object detection and semantic segmentation method based on deep convolution network
A RGB image and convolutional network technology, applied in the field of deep learning and machine vision, can solve problems such as slowness, complexity, and slow use speed
- Summary
- Abstract
- Description
- Claims
- Application Information
AI Technical Summary
Problems solved by technology
Method used
Image
Examples
Embodiment Construction
[0024] The present invention will be further described in detail below in conjunction with the accompanying drawings and embodiments.
[0025] The present invention will be described from the following aspects: fusion of RGB images and depth images, modified NMS, model training and experimental results.
[0026]The object detection and semantic segmentation method of RGB-D images based on deep convolutional network includes the following steps:
[0027] First, according to the above method, the RGB image and the depth image are fused into an HHG image;
[0028] Second, train the object detection system model;
[0029] There are three training methods of Faster-RCNN: one is Alternating Training, the other is Approximate Joint Training, and the third is Non-approximate Joint Training. This method uses an alternate training scheme. The idea of the alternate training scheme is to make the regional scheme network and Fast-RCNN share the convolutional layer parameters, and fine-...
PUM
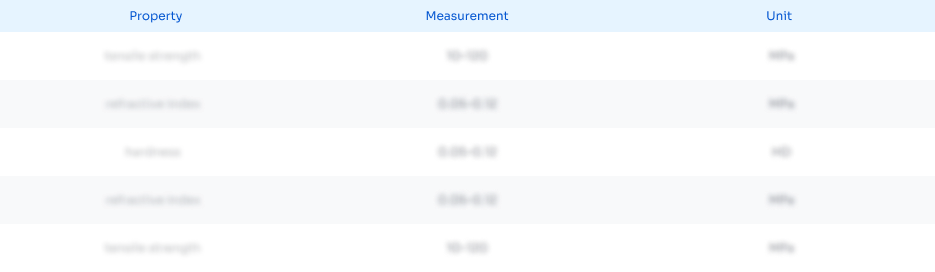
Abstract
Description
Claims
Application Information
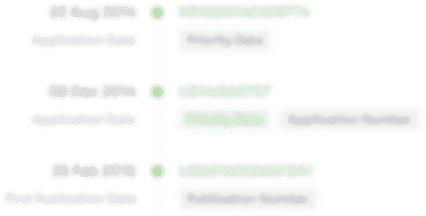
- R&D Engineer
- R&D Manager
- IP Professional
- Industry Leading Data Capabilities
- Powerful AI technology
- Patent DNA Extraction
Browse by: Latest US Patents, China's latest patents, Technical Efficacy Thesaurus, Application Domain, Technology Topic, Popular Technical Reports.
© 2024 PatSnap. All rights reserved.Legal|Privacy policy|Modern Slavery Act Transparency Statement|Sitemap|About US| Contact US: help@patsnap.com