Method for identifying human face under non-restricted condition based on LBP and deep learning
A face recognition, non-restricted technology, applied in the field of face recognition, can solve the problems of unfavorable feature expression, ignoring the local structure of the image, difficult to learn the local features of the face image, etc., and achieve high recognition rate and good recognition effect
- Summary
- Abstract
- Description
- Claims
- Application Information
AI Technical Summary
Problems solved by technology
Method used
Image
Examples
Embodiment 1
[0023] LFW face database experiment: The face images in LFW are collected from the Internet with the standard face detector Viola-Jones, including 13233 images of 5749 people. Among them, the number of images for 1680 persons was greater than or equal to two, and the other 4069 persons had only one image. The image resolution is 250×250, and the face images are mainly in color and contain a small amount of grayscale images. LFW is mainly used for face recognition under unrestricted conditions. This library can fully express changes in face images under real conditions, such as changes in posture, illumination, occlusion, expression, background, race, gender, etc. This application selects people whose number of images is greater than or equal to 20 as the experimental subjects, including 62 people and a total of 3023 images. Each person randomly selects 5 images as training samples and the rest as testing samples.
[0024] 1) Algorithm performance when the number of hidden un...
Embodiment 2
[0038] Yale face database experiment: Yale face database has 15 people, each with 11 images, a total of 165 images, the image gray level is 256, and the resolution is 243×320. Each person has 6 different expressions, 3 different lighting, and the image has the difference between wearing glasses and not wearing glasses. In the experiment, each person randomly selects 5 images as training samples, and the rest as testing samples.
[0039] Table 4: Correct recognition rate for different hidden units on the Yale library
[0040]
[0041] It can be seen from Table 4 that when there are fewer hidden units, the same deep network cannot accurately learn the category information of face images under the restricted conditions. With the increase of the number of hidden units, the network learns more and more features, and the number of hidden units When it is 5000, the face image features learned by the network are more discriminative.
[0042] Table 5: Correct recognition rate of...
Embodiment 3
[0047] Table 6: Correct recognition rate for different hidden units on the Yale-B library
[0048]
[0049] It can be seen from Table 6 that the algorithm in this paper has strong robustness to illumination changes.
[0050] Table 7: Correct recognition rate of different algorithms on the Yale-B library
[0051]
[0052] It can be seen from Table 7 that the algorithm of this application is equivalent to PCA, SVM, MSR (muitiscale retinex), SQI (self-quotient image), LBP, DBN and other algorithms in the recognition rate of subset 2 with little change in illumination, but when the illumination becomes complex , as shown in the experimental results on subset 3, the algorithm of this application can maintain a relatively stable recognition rate, while the recognition rate of the traditional algorithm drops sharply, which further shows that the algorithm of this paper is more robust to illumination.
[0053] Summary: From the results of the above examples, it can be seen th...
PUM
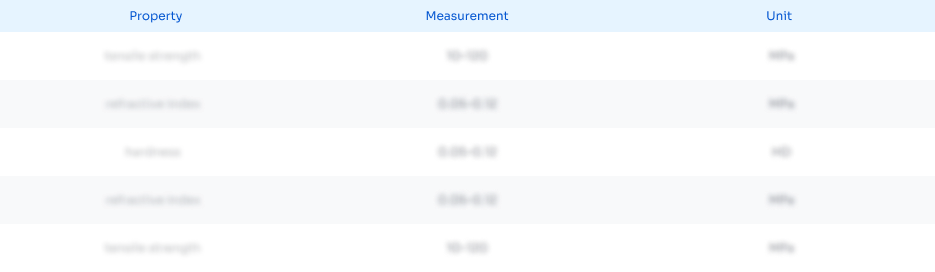
Abstract
Description
Claims
Application Information
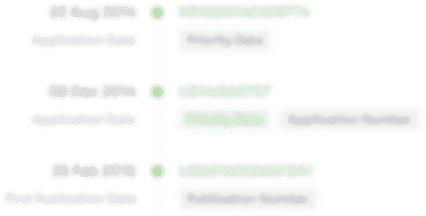
- R&D Engineer
- R&D Manager
- IP Professional
- Industry Leading Data Capabilities
- Powerful AI technology
- Patent DNA Extraction
Browse by: Latest US Patents, China's latest patents, Technical Efficacy Thesaurus, Application Domain, Technology Topic, Popular Technical Reports.
© 2024 PatSnap. All rights reserved.Legal|Privacy policy|Modern Slavery Act Transparency Statement|Sitemap|About US| Contact US: help@patsnap.com