Medical image segmentation method based on correlation matrix self-learning and explicit rank constraint
An association matrix, medical image technology, applied in the field of computer vision, can solve the problem of discount of segmentation effect, and achieve the effect of satisfying accuracy and obvious diagonal structure
- Summary
- Abstract
- Description
- Claims
- Application Information
AI Technical Summary
Problems solved by technology
Method used
Image
Examples
Embodiment Construction
[0015] The present invention will be further described below.
[0016] The specific method includes the following steps (such as figure 1 shown):
[0017] Step 1: The CT machine takes the CT image of the human body, obtains the DICOM file, analyzes and reads the pixel data of the image. Modify the gray level of the image data, and then perform the histogram equalization operation to enhance the contrast of the image area components and make the image details clearer. Filter the target segmentation area, use the human-computer interaction function roipoly in MATLAB to formulate the polygon of the target area, and use the filter to filter until the CT image achieves the most suitable segmentation effect.
[0018] Step 2, divide the preprocessed image pixel data into n pixel blocks x in sequence, each pixel block x contains m pixel elements, and merge them into an m×n dimensional matrix X=[x 1 ,x 2 ,...,x n ]∈R m×n The input is based on the algorithm model of correlation ma...
PUM
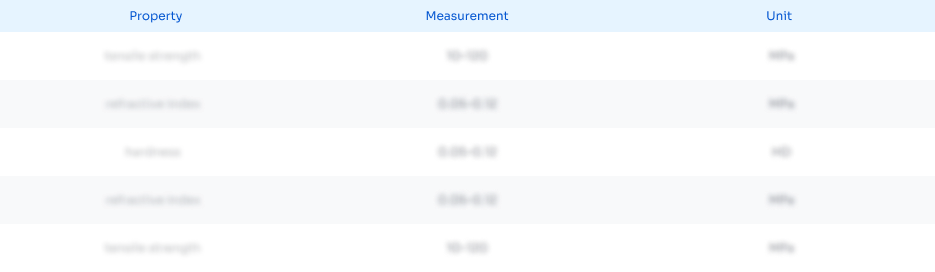
Abstract
Description
Claims
Application Information
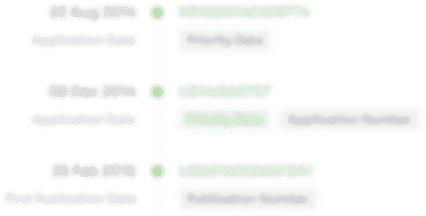
- R&D
- Intellectual Property
- Life Sciences
- Materials
- Tech Scout
- Unparalleled Data Quality
- Higher Quality Content
- 60% Fewer Hallucinations
Browse by: Latest US Patents, China's latest patents, Technical Efficacy Thesaurus, Application Domain, Technology Topic, Popular Technical Reports.
© 2025 PatSnap. All rights reserved.Legal|Privacy policy|Modern Slavery Act Transparency Statement|Sitemap|About US| Contact US: help@patsnap.com