Fault detection method based on particle swam optimization kernel independent-component analysis model
A technology of nuclear independent element analysis and particle swarm optimization, applied in electrical testing/monitoring, testing/monitoring control systems, instruments, etc., can solve the problem of inability to effectively mine useful information of nonlinear process data, time-consuming, and increase calculation time-consuming And other issues
- Summary
- Abstract
- Description
- Claims
- Application Information
AI Technical Summary
Problems solved by technology
Method used
Image
Examples
Embodiment Construction
[0032] The method of the present invention will be described in detail below in conjunction with the accompanying drawings.
[0033] Such as figure 1 As shown, the present invention provides a kind of fault detection method based on the particle swarm optimization nuclear independent element analysis model, and the specific implementation steps of the method are as follows:
[0034] Step 1: From the historical database of the production process, find out the sampling data of the process object in the normal operation state to form a data matrix X∈R n×m , and standardize each variable in X to get a new matrix with mean 0 and standard deviation 1 Among them, n is the number of training samples, m is the number of process measurement variables, superscript T represents the transposition of matrix or vector, R is the set of real numbers, R n×m Represents an n×m-dimensional real number matrix.
[0035] Step 2: After setting the kernel function parameter c=5m, calculate the kerne...
PUM
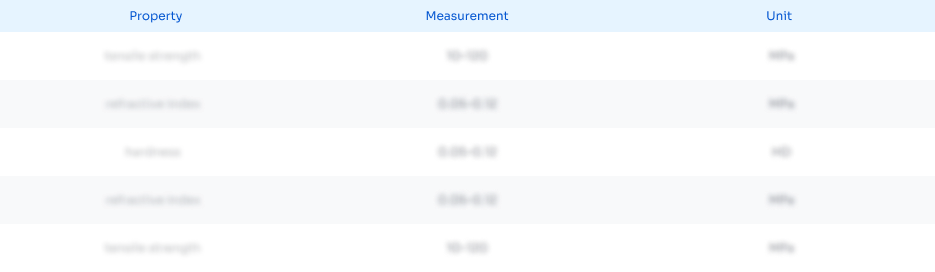
Abstract
Description
Claims
Application Information
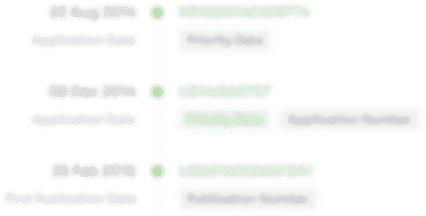
- R&D Engineer
- R&D Manager
- IP Professional
- Industry Leading Data Capabilities
- Powerful AI technology
- Patent DNA Extraction
Browse by: Latest US Patents, China's latest patents, Technical Efficacy Thesaurus, Application Domain, Technology Topic, Popular Technical Reports.
© 2024 PatSnap. All rights reserved.Legal|Privacy policy|Modern Slavery Act Transparency Statement|Sitemap|About US| Contact US: help@patsnap.com