Traffic flow rate prediction method based on road clustering and two-layer two-way LSTM (long short-term memory)
A technology of traffic flow and prediction method, which is applied in traffic flow detection, road vehicle traffic control system, traffic control system, etc., to achieve the effect of increasing speed, improving accuracy, and avoiding communication and synchronization
Inactive Publication Date: 2017-09-12
凯习(北京)信息科技有限公司
View PDF6 Cites 45 Cited by
- Summary
- Abstract
- Description
- Claims
- Application Information
AI Technical Summary
Problems solved by technology
Aiming at the current situation that the accuracy and speed of traffic flow prediction by neural network are difficult to improve and some deficiencies in existing designs, a traffic flow prediction method based on road clustering and double-layer bidirectional LSTM deep neural network model is proposed. Using the information hidden in the data and realizing the multiplexing of important information, the correlation clustering of roads improves the accuracy of traffic flow prediction, and avoids the communication and synchronization between nodes of the neural network model during cluster training, which improves the traffic flow prediction accuracy. Speed of cluster training
Method used
the structure of the environmentally friendly knitted fabric provided by the present invention; figure 2 Flow chart of the yarn wrapping machine for environmentally friendly knitted fabrics and storage devices; image 3 Is the parameter map of the yarn covering machine
View moreImage
Smart Image Click on the blue labels to locate them in the text.
Smart ImageViewing Examples
Examples
Experimental program
Comparison scheme
Effect test
Embodiment Construction
the structure of the environmentally friendly knitted fabric provided by the present invention; figure 2 Flow chart of the yarn wrapping machine for environmentally friendly knitted fabrics and storage devices; image 3 Is the parameter map of the yarn covering machine
Login to View More PUM
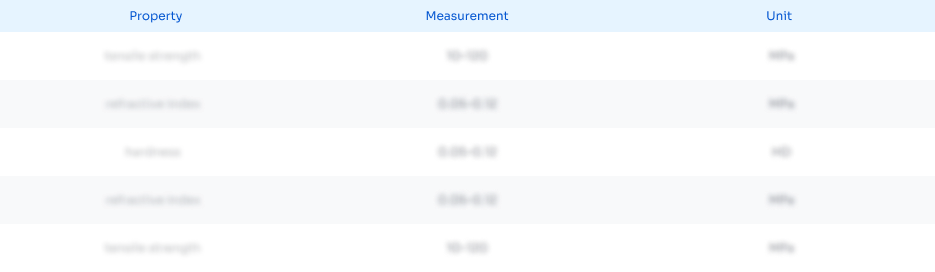
Abstract
The invention discloses a traffic flow rate prediction method based on road clustering and two-layer two-way LSTM (long short-term memory). The method comprises the following steps of (1) providing a mode of using peripheral equalization on a loss value when the training data has a missing value, so that the missing data is filled; the prediction precision is improved; (2) providing a method of performing relevance clustering on the road according to the historical flow rate data; dividing the road into a plurality of groups; simultaneously utilizing the time information and the space information in the data preprocessing stage for improving the prediction precision; (3) designing a two-layer two-way LSTM deep neural network model for improving the prediction precision of the model; (4) providing a method of performing mass training and test on the network model; accelerating the training and test speed of the neural network model; (5) providing a multi-model fusion method for improving the prediction precision. The method provided by the invention has the advantages that the prediction speed and the prediction precision of the deep neural network model in an aspect of traffic flow rate prediction are accelerated and improved at the same time.
Description
A Traffic Flow Prediction Method Based on Road Clustering and Bidirectional LSTM technical field The present invention relates to technologies such as deep learning, traffic flow prediction, neural network design, and cluster training of neural network models, especially a deep neural network model based on road clustering and double-layer LSTM (Long Short-Term Memory, long-term short-term memory) The traffic flow forecasting method has guiding significance for improving the accuracy and speed of traffic flow forecasting. Background technique With the advent of the era of big data, real-time traffic network data in big cities is gradually increasing. As one of the most critical applications in the current popular unmanned driving technology, artificial intelligence traffic prediction can make reasonable predictions on traffic conditions after considering the relationship between time and space. , to help vehicles choose the most suitable route, especially in the case of ur...
Claims
the structure of the environmentally friendly knitted fabric provided by the present invention; figure 2 Flow chart of the yarn wrapping machine for environmentally friendly knitted fabrics and storage devices; image 3 Is the parameter map of the yarn covering machine
Login to View More Application Information
Patent Timeline
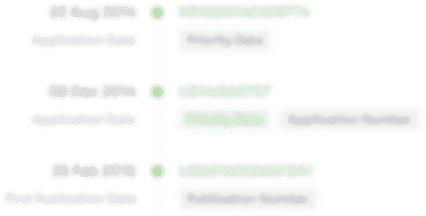
Patent Type & Authority Applications(China)
IPC IPC(8): G08G1/01G08G1/065G06N3/08G06K9/62
CPCG06N3/08G08G1/0129G08G1/065G06F18/23
Inventor 杨海龙黄秋宇栾钟治李云春
Owner 凯习(北京)信息科技有限公司
Features
- R&D
- Intellectual Property
- Life Sciences
- Materials
- Tech Scout
Why Patsnap Eureka
- Unparalleled Data Quality
- Higher Quality Content
- 60% Fewer Hallucinations
Social media
Patsnap Eureka Blog
Learn More Browse by: Latest US Patents, China's latest patents, Technical Efficacy Thesaurus, Application Domain, Technology Topic, Popular Technical Reports.
© 2025 PatSnap. All rights reserved.Legal|Privacy policy|Modern Slavery Act Transparency Statement|Sitemap|About US| Contact US: help@patsnap.com