An artificial intelligence algorithm model for building a personalized recommendation engine
An artificial intelligence and algorithm model technology, applied in the Internet field, can solve problems such as unstable actual effect, and achieve the effect of efficient hardware utilization, easy expansion, and good performance
- Summary
- Abstract
- Description
- Claims
- Application Information
AI Technical Summary
Problems solved by technology
Method used
Image
Examples
specific Embodiment
[0038] An artificial intelligence algorithm model for building a personalized recommendation engine, including: its logistic regression predicts the probability of occurrence of a target event through linear weighting and sigmoid transformation:
[0039] 1) Model description
[0040]
[0041] Among them, y is the target variable value {0,1}, x is the feature vector, and w is the model parameter.
[0042] We introduce the latent variable z, the membership degree (softmax) of the sample x to the model z, and the corresponding model parameter w_z:
[0043]
[0044]
[0045]
[0046] 2) Model training
[0047] Due to the introduction of latent variables, we use the EM algorithm to train the model. For the dataset, the log-likelihood function of the model is as follows:
[0048]
[0049] For the E-step of the EM algorithm, we need to make the equation state of the last inequality above true, that is, let the part after log become a constant:
[0050]
[0051] ...
experiment example
[0105] Taking the game product id tag for the user as an example, it is assumed that the following game is a page game game.
[0106] 1. First clarify the format of the output result of the labeling:
[0107] userid->[gameid1,gameid2,gameid3,...]
[0108] 2. Each page game game has an official website, the advertisement has a landing page, and the game type (strategy, action, legend, beauty, etc.)
[0109] 3. For users, assume that there are game types that users like (data produced by other teams)
[0110] 4. In addition, we may collect these data:
[0111] Duration of staying on the game landing page: short, medium, long; number of game landing page views; game theme official website home page visits; game registration / login times,
[0112] 5. Each action has an occurrence time, divided into 7 time windows: within 1 day, 1-3 days, 3-7 days, 7-15 days, 15-30 days, 30-60 days, 60-90 days ,
[0113] 6. Construct the user's actions on the game, the time of occurrence and whet...
PUM
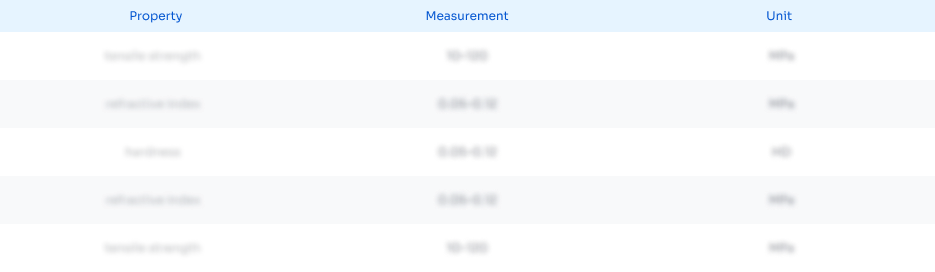
Abstract
Description
Claims
Application Information
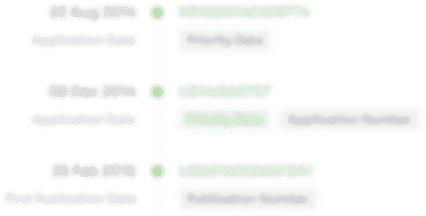
- Generate Ideas
- Intellectual Property
- Life Sciences
- Materials
- Tech Scout
- Unparalleled Data Quality
- Higher Quality Content
- 60% Fewer Hallucinations
Browse by: Latest US Patents, China's latest patents, Technical Efficacy Thesaurus, Application Domain, Technology Topic, Popular Technical Reports.
© 2025 PatSnap. All rights reserved.Legal|Privacy policy|Modern Slavery Act Transparency Statement|Sitemap|About US| Contact US: help@patsnap.com