Stock transaction behavior prediction method based on bi-clustering excavation and Naive Bayes and AdaBoost
A forecasting method and stock trading technology, applied in forecasting, instrumentation, finance, etc., can solve problems such as difficulty in stock forecasting, instability, etc., and achieve the effects of pertinence, efficiency improvement, and reliability assurance
- Summary
- Abstract
- Description
- Claims
- Application Information
AI Technical Summary
Problems solved by technology
Method used
Image
Examples
Embodiment 1
[0045] figure 1 It is a flow chart of the stock trading behavior prediction method based on bicluster mining, naive Bayesian and AdaBoost disclosed by the present invention, as figure 1 As shown, the stock trading prediction method disclosed in this embodiment specifically includes the following steps:
[0046] S1. Calculate the future rate of return FRR on the i-th trading day in the historical stock data i .
[0047] Select the stock data for a period of time as the training set for stock forecasting, and calculate the stock raw data (including opening price, highest price, lowest price, closing price and trading volume) corresponding to each trading day according to different technical index formulas. At the same time, calculate the future rate of return FRR corresponding to each trading day. The specific steps are as follows:
[0048] S11, select the stock historical data of m days as the training sample of prediction model, as the data set of biclustering mining;
[...
Embodiment 2
[0077] The present embodiment selects specific stock—Apple Corporation (AAPL), and adopts the stock trading behavior prediction method based on bicluster mining and naive Bayesian and AdaBoost to predict the price change trend of the stock, specifically including the following steps:
[0078]1) Select the stock data (opening price, highest price, lowest price, closing price and trading volume) of Apple Inc. (AAPL) for m=365 days as the training set of the stock prediction model, and at the same time as the data set for mining stock information. Select 33 stock technical index values as the parameter indicators of each trading day, and calculate the future rate of return FRR of each trading day at the same time. The specific calculation formula is as follows:
[0079] For the i-th trading day and the following t trading days, the average closing price corresponding to the i-th trading day is: Among them, ACP i Indicates the average closing price of the i-th trading day, CP...
PUM
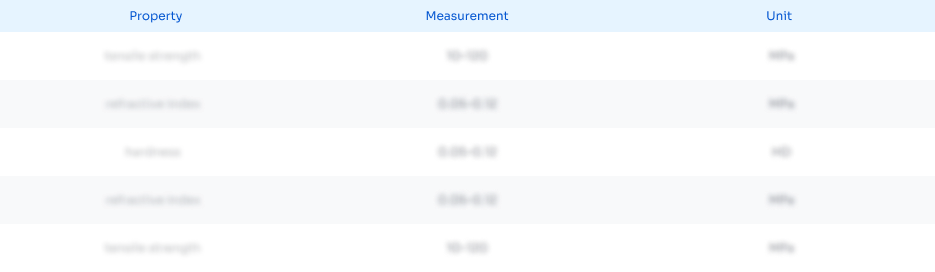
Abstract
Description
Claims
Application Information
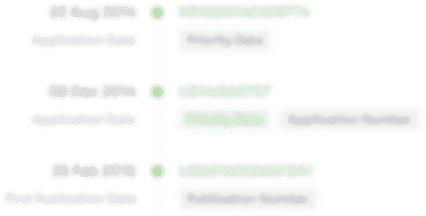
- R&D Engineer
- R&D Manager
- IP Professional
- Industry Leading Data Capabilities
- Powerful AI technology
- Patent DNA Extraction
Browse by: Latest US Patents, China's latest patents, Technical Efficacy Thesaurus, Application Domain, Technology Topic.
© 2024 PatSnap. All rights reserved.Legal|Privacy policy|Modern Slavery Act Transparency Statement|Sitemap