Fabric defect detection method based on depth neural network
A deep neural network and defect detection technology, applied in the field of image processing, can solve problems such as lack of adaptability, increased method complexity, and decreased recognition rate, achieving good adaptability, high timeliness, and excellent performance
- Summary
- Abstract
- Description
- Claims
- Application Information
AI Technical Summary
Problems solved by technology
Method used
Image
Examples
Embodiment 1
[0034] Embodiment one: see figure 1 As shown, a fabric defect detection method based on a deep neural network includes the following steps:
[0035] 1. During the fabric production process, the fabric image is taken from the fabric rolling machine by an industrial line scan camera as an experimental sample and sent to the computer.
[0036] 2. Divide the collected image from 4096*4096 pixels into experimental samples of 128*128 pixels, use image transformation and add salt and pepper noise, perform data enhancement on a part of the original image, and use the enhanced fabric image as training samples, the training samples contain normal and defect images of different fabrics. Calibrate the image, '0' represents a normal sample, and '1' represents a defective sample.
[0037] 3. see figure 2 As shown, the deep neural network is designed, and the structure diagram of the deep neural network is shown in Figure II As shown, it includes one input layer, three convolutional la...
PUM
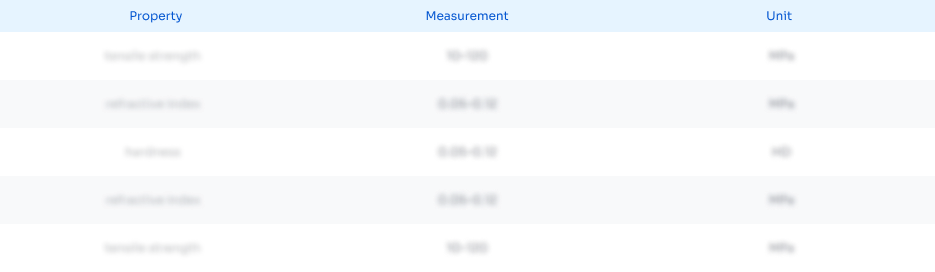
Abstract
Description
Claims
Application Information
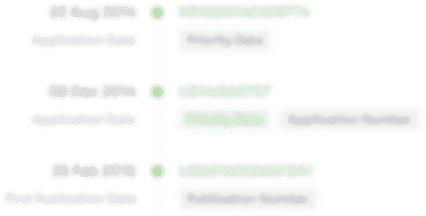
- R&D
- Intellectual Property
- Life Sciences
- Materials
- Tech Scout
- Unparalleled Data Quality
- Higher Quality Content
- 60% Fewer Hallucinations
Browse by: Latest US Patents, China's latest patents, Technical Efficacy Thesaurus, Application Domain, Technology Topic, Popular Technical Reports.
© 2025 PatSnap. All rights reserved.Legal|Privacy policy|Modern Slavery Act Transparency Statement|Sitemap|About US| Contact US: help@patsnap.com