Air quality prediction method based on ensemble extreme learning machine
An extreme learning machine and air quality technology, applied in the field of air quality prediction based on the integrated extreme learning machine, can solve the problem that the multi-layer perceptron is easy to fall into the local extreme value, so as to improve the generalization ability, improve the prediction accuracy and generalization. Ability-enhancing effect
- Summary
- Abstract
- Description
- Claims
- Application Information
AI Technical Summary
Problems solved by technology
Method used
Image
Examples
Example Embodiment
[0049] Taking air quality prediction as an example, the following is a detailed description of the present invention with examples and drawings.
[0050] The invention uses a PC and requires a GPU with sufficient computing power to accelerate training. Such as figure 1 As shown, the specific steps of an air quality prediction method based on an extreme learning machine provided by the present invention are as follows:
[0051] Step 1. Construct the input data and output data of the model
[0052] The input vector X = {x 1 , X 2 ,...X i ,...X n } And the output vector Y = {y 1 , Y 2 ,...Y i ,...Y n }. Each variable in X represents a factor related to air quality, such as wind, wind direction, and sulfur dioxide concentration. X takes the historical data of air quality related factors at the current moment, and can also add the forecast value of the weather forecast. Y is the expected output, where each variable represents the air quality for every hour in the next 24 hours at the c...
PUM
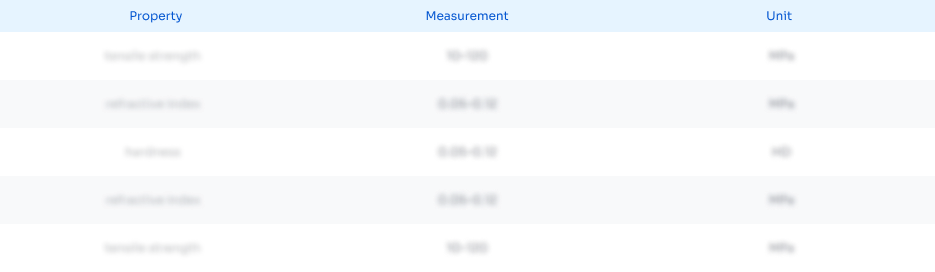
Abstract
Description
Claims
Application Information
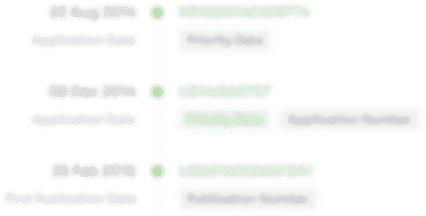
- R&D Engineer
- R&D Manager
- IP Professional
- Industry Leading Data Capabilities
- Powerful AI technology
- Patent DNA Extraction
Browse by: Latest US Patents, China's latest patents, Technical Efficacy Thesaurus, Application Domain, Technology Topic.
© 2024 PatSnap. All rights reserved.Legal|Privacy policy|Modern Slavery Act Transparency Statement|Sitemap