Fault detection method and system of non-Gaussian industrial process
A fault detection and industrial process technology, applied in the direction of electrical program control, comprehensive factory control, etc., can solve problems such as only considering a single data set, no better solution, limited kernel function parameter selection, etc.
- Summary
- Abstract
- Description
- Claims
- Application Information
AI Technical Summary
Problems solved by technology
Method used
Image
Examples
Embodiment 1
[0041] A non-Gaussian industrial process fault detection method, referring to figure 1 ,include:
[0042] The first step is to select a certain number of normal historical data sets, preprocess the data sets to remove the mean value, and use the canonical correlation analysis method to establish a residual generator. This step can be performed offline and includes:
[0043]Step A1, using the historical database to select process data under normal operating conditions, using Indicates two variable data sets of the process, where l is the number of measured variables in vector u, m is the number of measured variables in vector y, and N is the number of independent sampling points; then u(i), i=1,...,N represent the vector The measured value of the l variable in u at the i-th sampling time, y(i), i=1,..., N represents the measured value of the m variables in the vector y at the i-th sampling time.
[0044] Step A2. Perform de-mean processing on the data set; respectively perf...
Embodiment 2
[0097] As a degraded implementation of the above-mentioned embodiment 1, in the above-mentioned detection process, it is possible to simply rely on the first residual generator or the second residual generator for detection. On the premise of reducing the amount of related data processing, the accuracy is compared to The combined detection method of the first and second residual generators degrades slightly.
[0098] The non-Gaussian industrial process fault detection method disclosed in this embodiment (can refer to the above-mentioned embodiment 1), including:
[0099] The first step is to select a certain number of normal historical data sets, preprocess the data sets to remove the mean value, and use the canonical correlation analysis method to establish a residual generator; specifically include:
[0100] Step A1, using the historical database to select process data under normal operating conditions, using Indicates two variable data sets of the process, where l is the ...
Embodiment 3
[0122] This embodiment is similar to the above-mentioned embodiment 2, and discloses a non-Gaussian industrial process fault detection method (can refer to the above-mentioned embodiment 1), including:
[0123] The first step is to select a certain number of normal historical data sets, preprocess the data sets to remove the mean value, and use the canonical correlation analysis method to establish a residual generator; specifically include:
[0124] Step A1, using the historical database to select process data under normal operating conditions, using Indicates two variable data sets of the process, where l is the number of measured variables in vector u, m is the number of measured variables in vector y, and N is the number of independent sampling points; then u(i), i=1,...,N represent the vector The measured value of the l variable in u at the i-th sampling moment, y(i), i=1,..., N represents the measured value of the m variable in the vector y at the i-th sampling moment; ...
PUM
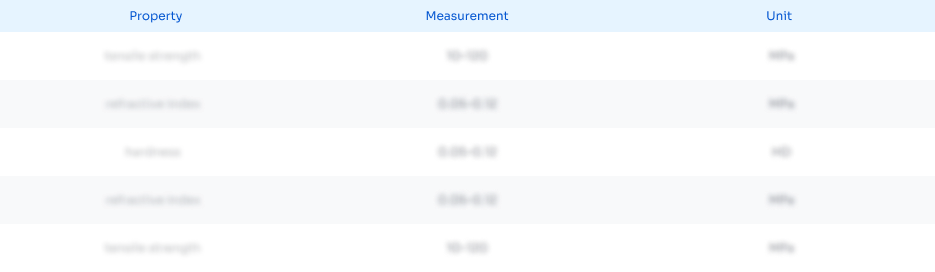
Abstract
Description
Claims
Application Information
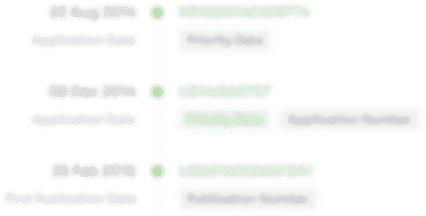
- R&D Engineer
- R&D Manager
- IP Professional
- Industry Leading Data Capabilities
- Powerful AI technology
- Patent DNA Extraction
Browse by: Latest US Patents, China's latest patents, Technical Efficacy Thesaurus, Application Domain, Technology Topic, Popular Technical Reports.
© 2024 PatSnap. All rights reserved.Legal|Privacy policy|Modern Slavery Act Transparency Statement|Sitemap|About US| Contact US: help@patsnap.com