A Quality Control Method for Crowdsourcing Classification Data Based on Self-paced Learning
A quality control method and classification data technology, applied in the field of crowdsourcing classification data quality control based on self-paced learning, can solve problems such as providing errors, random provision, uselessness, etc., to reduce the expenditure of crowdsourcing tasks and achieve high accuracy Effect
- Summary
- Abstract
- Description
- Claims
- Application Information
AI Technical Summary
Problems solved by technology
Method used
Image
Examples
Embodiment
[0024] The self-paced learning crowdsourcing classification data quality control method is generally divided into two parts. The first part is the data collection stage, which allows labelers to arbitrarily select topics for labeling. For the marked objects that the worker does not want to label or is not sure about, the worker can choose to skip. There is also no limit on the number of worker annotations, so the resulting annotation data may be very unbalanced and sparse. The second part is the discovery of real labels. This part is iteratively executed by selecting labels and estimating hidden real labels to obtain more accurate real labels and the real capabilities of workers.
[0025] (1) Data collection stage
[0026] In the data collection phase, the Figure 1 The method of user interaction, when marked by this method, the user can skip the question, and there is no limit to the user's answer to the least question, so that the user can answer at any time and stop at an...
PUM
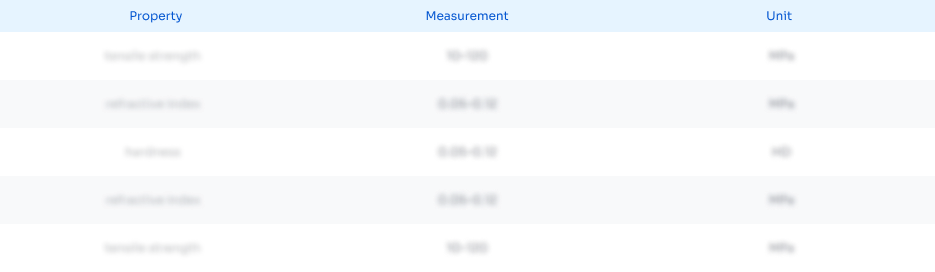
Abstract
Description
Claims
Application Information
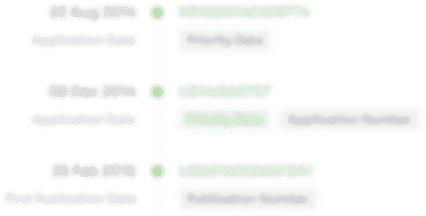
- R&D
- Intellectual Property
- Life Sciences
- Materials
- Tech Scout
- Unparalleled Data Quality
- Higher Quality Content
- 60% Fewer Hallucinations
Browse by: Latest US Patents, China's latest patents, Technical Efficacy Thesaurus, Application Domain, Technology Topic, Popular Technical Reports.
© 2025 PatSnap. All rights reserved.Legal|Privacy policy|Modern Slavery Act Transparency Statement|Sitemap|About US| Contact US: help@patsnap.com