Image super-resolution reconstruction method based on sparse autoencoder network and extremely fast learning
A sparse self-encoding and extremely fast learning technology, applied in the field of image super-resolution reconstruction based on sparse autoencoder network and extremely fast learning, can solve the problems of inaccurate feature extraction, missing neighbors, inaccurate reconstruction, etc., and achieve details and texture The effect of good information, improved accuracy, and enhanced versatility
- Summary
- Abstract
- Description
- Claims
- Application Information
AI Technical Summary
Problems solved by technology
Method used
Image
Examples
Embodiment 1
[0032] The present invention is an image super-resolution reconstruction method based on sparse autoencoder network and extremely fast learning, see figure 1 , the present invention includes the following steps to image super-resolution reconstruction:
[0033] Step 1: Input the training sample image pair, and divide the low-resolution training sample image into the size of The low-resolution training example image patches of and pulled into a vector Segment the high-resolution training example image into patches with the low-resolution training example image in the same way The corresponding size is High-resolution training example image patches of and pulled into a vector Where n represents the dimension of the low-resolution training sample image block vector, N represents the scale of high-resolution and low-resolution training sample image blocks, and s represents the upsampling multiple;
[0034] Step 2: Convert low-resolution training example image patch vector...
Embodiment 2
[0044] The image super-resolution reconstruction method based on sparse autoencoder network and extremely fast learning is the same as that in Embodiment 1, refer to the appendix figure 1 , the specific steps of the image super-resolution reconstruction method based on sparse autoencoder network and extremely fast learning of the present invention include:
[0045] 1. An image super-resolution reconstruction method based on representation learning and neighborhood constraint embedding (an image super-resolution reconstruction method based on sparse autoencoder network and extremely fast learning), characterized in that it includes the following steps:
[0046] Step 1: Input training sample image pairs, see figure 2 , image 3 , split the low-resolution training sample image into a size of The low-resolution training example image patches of and pulled into a vector Segment the high-resolution training example image into patches with the low-resolution training example im...
Embodiment 3
[0058] The image super-resolution reconstruction method based on sparse autoencoder network and extremely fast learning is the same as that in Embodiment 1-2.
PUM
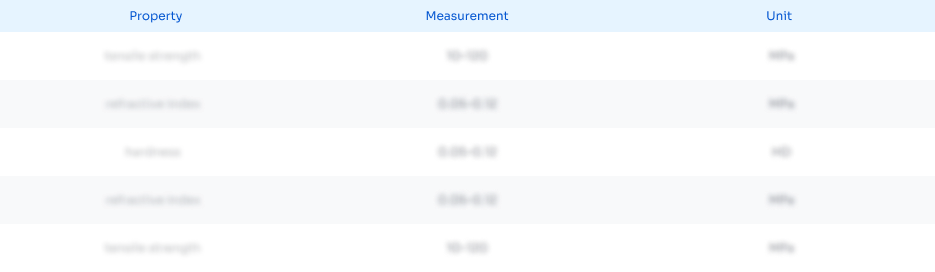
Abstract
Description
Claims
Application Information
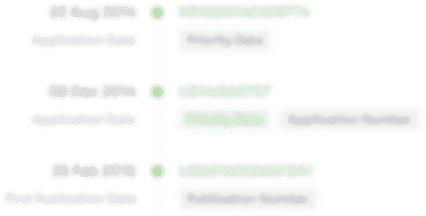
- R&D Engineer
- R&D Manager
- IP Professional
- Industry Leading Data Capabilities
- Powerful AI technology
- Patent DNA Extraction
Browse by: Latest US Patents, China's latest patents, Technical Efficacy Thesaurus, Application Domain, Technology Topic, Popular Technical Reports.
© 2024 PatSnap. All rights reserved.Legal|Privacy policy|Modern Slavery Act Transparency Statement|Sitemap|About US| Contact US: help@patsnap.com