Fuzzy neural network algorithm integrating classification and clustering into one body
A fuzzy neural network and clustering technology, which is applied in biological neural network models, neural learning methods, neural architectures, etc., can solve problems such as ambiguity, irrationality, and super-box overlap
- Summary
- Abstract
- Description
- Claims
- Application Information
AI Technical Summary
Problems solved by technology
Method used
Image
Examples
Embodiment Construction
[0070] Below in conjunction with specific embodiment, further illustrate the present invention.
[0071] The present invention provides a fuzzy neural network algorithm integrating "classification and clustering". , to cluster the sample set; (2) when the sample set is all marked, the subsequent learning process is mainly based on supervised learning, and the sample is classified; (3) when the sample is partly marked and partly unidentified marked, adopt the same learning method as (2), that is, the supervised learning method.
[0072] 1. Basic definition:
[0073] 1. Input vector
[0074] The input pattern of the ensemble learning model adopts the sequence pair of the following form: {X h , dh}.
[0075] in, represents the hth input pattern, is the low endpoint, is the high end. while d h ∈ {0, 1, 2, ... p} represents the category label of a certain class in the p+1 class, when d h = 0 means that the input sample is an unlabeled sample.
[0076] The hyperbox def...
PUM
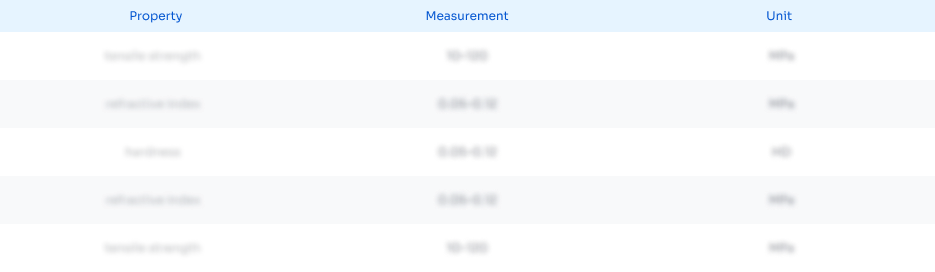
Abstract
Description
Claims
Application Information
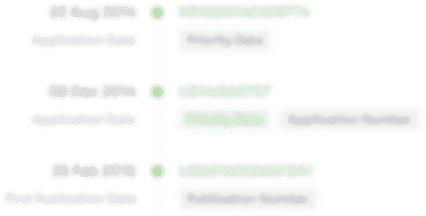
- R&D
- Intellectual Property
- Life Sciences
- Materials
- Tech Scout
- Unparalleled Data Quality
- Higher Quality Content
- 60% Fewer Hallucinations
Browse by: Latest US Patents, China's latest patents, Technical Efficacy Thesaurus, Application Domain, Technology Topic, Popular Technical Reports.
© 2025 PatSnap. All rights reserved.Legal|Privacy policy|Modern Slavery Act Transparency Statement|Sitemap|About US| Contact US: help@patsnap.com