Mutual information-kernel principal component analysis-Elman network-based medium-long-term runoff forecast method
A kernel principal component analysis and mutual information technology, which is applied in the field of medium and long-term runoff forecasting based on mutual information-kernel principal component analysis-Elman network, which can solve the influence of neural network model parameter prediction accuracy, differences, and non-optimal principal components, etc. question
- Summary
- Abstract
- Description
- Claims
- Application Information
AI Technical Summary
Problems solved by technology
Method used
Image
Examples
Embodiment Construction
[0092] The present invention will be further elaborated below in conjunction with the accompanying drawings and embodiments.
[0093] figure 1 It is an overall flowchart of the present invention. Taking the forecast of average annual runoff of Jinping I Hydropower Station reservoir as an example, according to the flow chart, it can be divided into six steps, as follows:
[0094] Step 1: Data Preprocessing
[0095] 1.1 Collect historical runoff data in the area to be predicted and meteorological and hydrological data that may be used as predictors. Commonly used meteorological and hydrological data include indicators such as atmospheric circulation characteristics, upper air pressure field, and sea surface temperature. The data used in this embodiment include the yearly average runoff data of the reservoir section of the Jinping I Hydropower Station from 1960 to 2011 and the monthly 74 circulation feature data from 1959 to 2010.
[0096] 1.2 Since it is forecasting the aver...
PUM
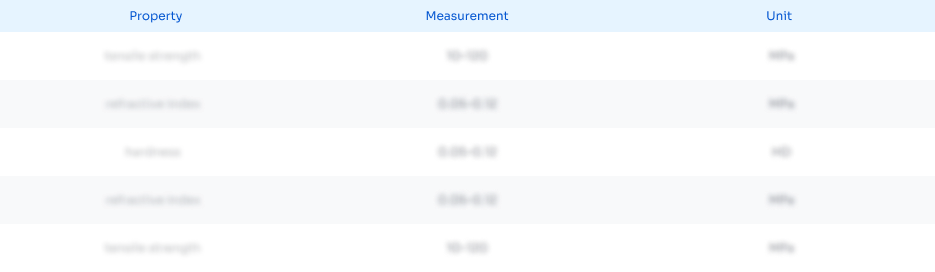
Abstract
Description
Claims
Application Information
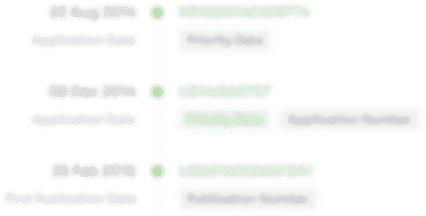
- R&D Engineer
- R&D Manager
- IP Professional
- Industry Leading Data Capabilities
- Powerful AI technology
- Patent DNA Extraction
Browse by: Latest US Patents, China's latest patents, Technical Efficacy Thesaurus, Application Domain, Technology Topic.
© 2024 PatSnap. All rights reserved.Legal|Privacy policy|Modern Slavery Act Transparency Statement|Sitemap