Visual target retrieval method and system based on target detection
A target detection and retrieval system technology, applied in special data processing applications, instruments, electrical digital data processing, etc., can solve the problems of noise interference, poor practicability, noise, etc., to achieve the effect of improving average accuracy and speeding up retrieval speed
- Summary
- Abstract
- Description
- Claims
- Application Information
AI Technical Summary
Problems solved by technology
Method used
Image
Examples
Embodiment Construction
[0034] In view of the above problems, the present invention proposes a kind of attention mechanism based on detection and voting for image retrieval ( Figure 5 Shown), and the following specific examples, and in conjunction with the accompanying drawings described in detail as follows. The invention belongs to the image search technology based on deep learning. The whole technical framework of the present invention is as Figure 6 as shown, Figure 6 The middle and lower part corresponds to the offline network training stage, which is the frame diagram of Faster R-CNN, which obtains a target detection model through IDF (inverse document frequency) weighted cross-entropy loss function training, which is the final target detection model below; Figure 6 The upper middle part corresponds to the online feature extraction stage, which uses the final target detection model to extract the feature vector of the convolutional layer of the picture to be retrieved, and aggregates the ...
PUM
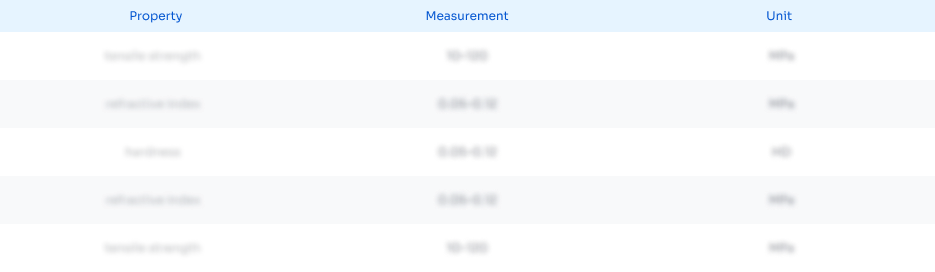
Abstract
Description
Claims
Application Information
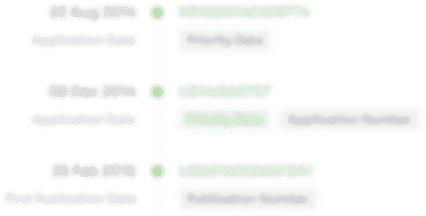
- R&D Engineer
- R&D Manager
- IP Professional
- Industry Leading Data Capabilities
- Powerful AI technology
- Patent DNA Extraction
Browse by: Latest US Patents, China's latest patents, Technical Efficacy Thesaurus, Application Domain, Technology Topic, Popular Technical Reports.
© 2024 PatSnap. All rights reserved.Legal|Privacy policy|Modern Slavery Act Transparency Statement|Sitemap|About US| Contact US: help@patsnap.com