Local scale parameter, entropy and cosine similarity-based spectral feature selection method
A cosine similarity and scale parameter technology, which is applied in the fields of electrical digital data processing, special data processing applications, unstructured text data retrieval, etc., can solve the influence of outliers, and the uniform scale parameter σ cannot completely and accurately reflect the data distribution information And other issues
- Summary
- Abstract
- Description
- Claims
- Application Information
AI Technical Summary
Problems solved by technology
Method used
Image
Examples
Embodiment
[0197] The present invention verifies the validity of four newly proposed feature selection methods based on spectral clustering, and compares the performance of the four spectral feature selection methods with the multi-category cluster feature selection method MCFS and the Laplacian score feature selection method. To this end, the proposed four spectral feature selection methods FSSC_OE, FSSC_OC, FSSC_SE, FSSC_SC, and the comparison algorithm MCFS and Laplacian score feature selection methods are used to achieve feature selection, and the corresponding feature subsets are obtained. The training samples are used to construct the SVM classifier, and the accuracy, sensitivity, specificity and other indicators of the classifier are compared.
[0198] Using a 10-fold cross-validation experiment, first add samples of each class to 10 sample sets one by one (the initial sample set is empty), until each sample of this class is added, and the samples are evenly divided into 10 share....
PUM
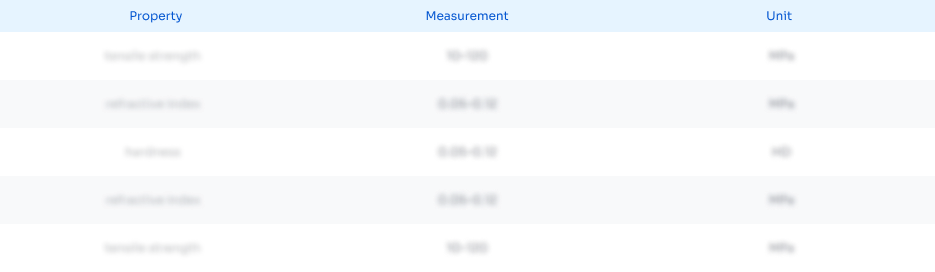
Abstract
Description
Claims
Application Information
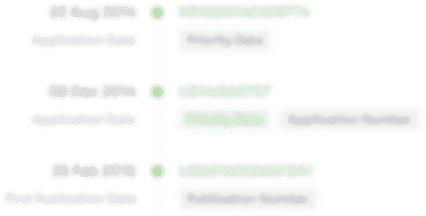
- R&D Engineer
- R&D Manager
- IP Professional
- Industry Leading Data Capabilities
- Powerful AI technology
- Patent DNA Extraction
Browse by: Latest US Patents, China's latest patents, Technical Efficacy Thesaurus, Application Domain, Technology Topic, Popular Technical Reports.
© 2024 PatSnap. All rights reserved.Legal|Privacy policy|Modern Slavery Act Transparency Statement|Sitemap|About US| Contact US: help@patsnap.com