Multi-task layered image retrieval method based on depth self-coding convolution neural network
A convolutional neural network and image retrieval technology, which is applied in the direction of biological neural network models, neural architectures, and special data processing applications, can solve the problems of slow large-scale image retrieval, accelerated retrieval speed, low level of automation and intelligence, etc. , to achieve the effect of meeting image retrieval requirements, speeding up retrieval, and reducing memory dependence
- Summary
- Abstract
- Description
- Claims
- Application Information
AI Technical Summary
Problems solved by technology
Method used
Image
Examples
Embodiment Construction
[0081] The following will clearly and completely describe the technical solutions in the embodiments of the present invention with reference to the drawings in the embodiments of the present invention.
[0082] refer to Figure 1 to Figure 6 , a multi-task hierarchical image retrieval method based on deep self-encoding convolutional neural network, such as Figure 6 As shown, the input retrieved image first passes through the shared module of the convolutional neural network, and then is sent to the interest module to screen out the position of the rough region of interest in the image, and then sent to the fast model based on the interest module for the secondary screening of the region of interest. The precise position of the target in the image can be obtained by visual segmentation detection and positioning. Through the method of deep learning, the sparse hash code of the entire image can be obtained for rough retrieval, and the region-aware semantic features of the maximu...
PUM
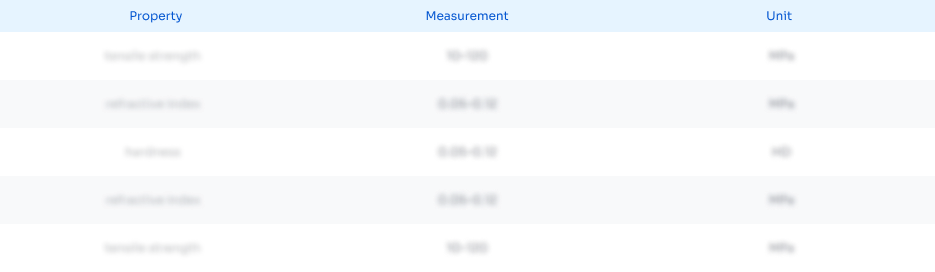
Abstract
Description
Claims
Application Information
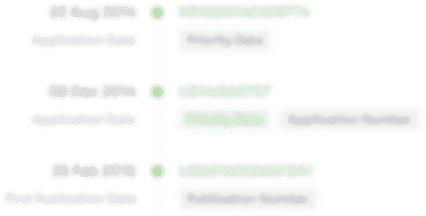
- R&D Engineer
- R&D Manager
- IP Professional
- Industry Leading Data Capabilities
- Powerful AI technology
- Patent DNA Extraction
Browse by: Latest US Patents, China's latest patents, Technical Efficacy Thesaurus, Application Domain, Technology Topic, Popular Technical Reports.
© 2024 PatSnap. All rights reserved.Legal|Privacy policy|Modern Slavery Act Transparency Statement|Sitemap|About US| Contact US: help@patsnap.com